Maria Dadarlat, Ph.D.
University of California, Berkeley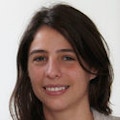
Maria Dadarlat was a postdoctoral researcher in the physiology department at the University of California, San Francisco (UCSF) and is now an assistant professor in the Department of Biomedical Engineering at Purdue University. She received her B.S. in biomedical engineering from Purdue University and in 2014 completed her Ph.D. in the UC Berkeley-UCSF Joint Graduate Program in Bioengineering.
She is interested in the basic, systems-level mechanisms of neural coding and plasticity and the application of these principles to neural prosthetics. Her thesis work concerned the latter topic, studying how to use electrical stimulation of primary somatosensory cortex to deliver artificial sensation. As a postdoctoral scholar in the lab of Michael Stryker at UCSF, she studied how neural encoding of vision is modulated by behavioral states.
Project: Dynamic encoding of visual stimuli in mouse cortex
When we move through the world, we change the patterns of incoming sensory information. For example, a runner takes in more visual information per second than a walker does. Interestingly, this change in behavior also changes the way neurons in visual areas of the brain respond to visual stimuli. It seems that when an animal is running, visual neurons enter a high-gain state, meaning they become more sensitive to incoming visual information. Yet, while we have some idea of how visual neurons enter the high-gain state, we have little idea as to why they do so. Furthermore, the existence of a high-gain state raises an interesting question: if neurons can respond differently to the same visual input, then how does the brain correctly identify objects across different behavioral states? To investigate these questions, this project plans to record from neurons in visual areas of a mouse’s brain while that mouse is either running or standing still on a spherical treadmill. By recording from those neurons, researchers will be able to reconstruct how well objects are represented in each of the two behavioral states. They expect the rate of information encoding to be faster when the animal is running because the rate of incoming visual information will be faster as well. To understand how the representation of objects remains constant across different states, they will also record from the neurons to which visual neurons send connections. By doing this, they can observe how these so-called downstream neurons represent visual objects when the neurons sending them information are in either a high-gain (running) or a low-gain (still) state. These results will shed light on the fundamental question of how an animal’s movements affect the processing of neural information.