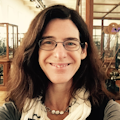
Adrienne Fairhall is a professor of physiology and biophysics at the University of Washington, director of the university’s computational neuroscience program, and co-director of its Institute for Neuroengineering. She obtained an honors degree in theoretical physics from the Australian National University and a Ph.D. in statistical physics in the lab of Itamar Procaccia at the Weizmann Institute of Science. She received her postdoctoral training at the NEC Research Institute with Bill Bialek and at Princeton University with Michael J. Berry II. Fairhall has directed the Marine Biological Laboratory course Methods in Computational Neuroscience. Fairhall is a recipient of the Burroughs Wellcome Fund Career Award and the McKnight Scholar Award and was a 2013 Allen Distinguished Investigator.
Fairhall’s work focuses on dynamic neural computation, with an emphasis on the interplay between cellular and circuit dynamics and coding. She develops theoretical approaches to understanding processing in nervous systems. She collaborates closely with experimental labs to uncover algorithms of information processing in a variety of sensory systems, including the retina, the somatosensory cortex, and areas performing visual motion detection, and in a range of other systems, from single neurons to foraging mosquitoes to navigating primates. Fairhall is interested in general methods for building reduced models for neural computation in terms of relevant features from a complex input. The methods she is exploring start both from experimental data and from biophysical descriptions of neural dynamics. She aims to relate the functional models determined by spiking data directly with the underlying channel dynamics. The brain is a highly adaptive organ, assimilating and adjusting to changes in the environment on a multiplicity of temporal and spatial scales. Thus, the neural code of several systems has been shown to be adaptive to changes in the statistical distribution of the inputs. In her work, she is seeking to elucidate how such adaptation may be beneficial for neural information processing, and she is exploring potential mechanisms underlying adaptation to statistics at the level of single-neuron computation.
Projects:
Neural circuit dynamics underlying sequence and variability
Neural substrates of behavior and action selection in model organisms