Nabil Iqbal, Ph.D.
Professor, Durham University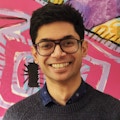
Nabil Iqbal is a professor of mathematical and theoretical physics at Durham University. He obtained his bachelor’s degree in physics and mathematics from Cornell University in 2006 and his Ph.D. from the Massachusetts Institute of Technology in 2011. He held postdoctoral appointments at the Kavli Institute of Theoretical Physics and the University of Amsterdam before joining Durham University as an assistant professor in 2017. He was promoted to associate professor in 2020 and full professor in 2023.
Iqbal works on a wide variety of topics in theoretical physics and has made contributions to problems in string theory, quantum field theory, gravity and statistical physics. One of his main interests is holographic duality: the idea that a quantum theory of gravity is exactly equivalent to a theory of particles living in one lower dimension. He has worked extensively on applications of holographic duality, connecting the physics of black holes to that of strongly correlated liquids and understanding holographic measures of quantum entanglement. He has also applied new symmetry principles from quantum field theory to the hydrodynamics of plasmas and the classification of the phases of matter. More recently, he has begun to study applications of machine learning to problems in statistical physics. In general, he is very interested in scientific problems that live at the intersections of different fields.
Research Blurb
Nabil Iqbal currently works as a theoretical high-energy physicist and has recently become interested in the interplay of machine learning and theoretical physics. He will use the Simons Foundation Pivot Fellowship to lay the foundations of a research program in machine learning, working on a project on geometric deep learning with his mentor Erik Bekkers at the Amsterdam Machine Learning Lab. He plans to build a new class of neural networks whose architecture encodes the symmetries of conformal invariance, a powerful symmetry group that appears in many places in nature. He hopes that this new class of networks will have applications to problems in computer vision and the characterization of three-dimensional data.