Hannah Lawrence
Ph.D. Student, Massachusetts Institute of Technology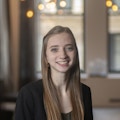
Hannah Lawrence first joined the Flatiron Institute in 2017 as a research intern at the Center for Computational Biology (CCB), where she developed FFT-based algorithms for MRI reconstruction. After graduating from Yale University in 2019, she spent the summer at Microsoft Research studying fast algorithms for Toeplitz matrices, before returning as a research analyst at the (then newly formed) Center for Computational Mathematics (CCM). There, she worked on developing deep learning methods for cryogenic electron microscopy and phase retrieval. Now a third-year Ph.D. student in machine learning at MIT, Hannah’s research interests include theoretical aspects of symmetry-constrained (equivariant) learning, such as understanding the representational power and sample complexity of such methods, as well as developing more practical tools for understanding and analyzing neural nets. She is excited by the promise of equivariant methods for scientific applications and looks forward to engaging more with applications during the remainder of her doctoral research. In 2019, Hannah received the Russell Henry Chittenden Prize from Yale. She is supported by a Hertz Fellowship and a National Science Foundation Graduate Fellowship.