2022 Flatiron Machine Learning X Science Summer School
- Application Deadline
- Program Dates
- to
- Please send all inquiries to
[email protected] - Summer School Organizers:
Miles Cranmer
Domenico Di Sante
Michael Eickenberg
Siavash Golkar
Shirley Ho
Natalie Sauerwald
The Flatiron Machine Learning X Science summer school starts with the motivation to introduce modern methods of statistical machine learning and inference to the scientific community. It was motivated by the observation that while many students are keen to learn about machine learning, and an increasing number of scientists want to apply machine learning methods to their research problems, very few machine learning courses teach state of the art methods with science applications in mind.
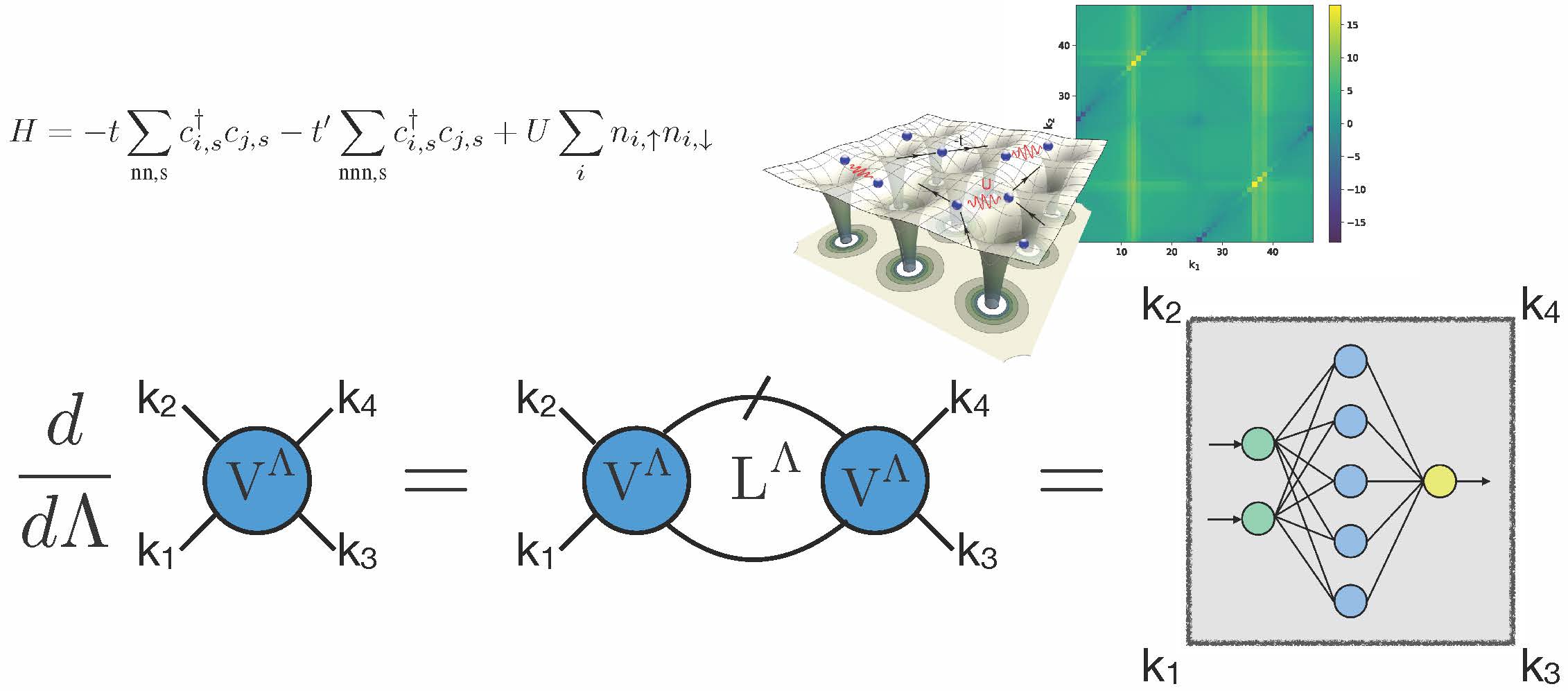
Flatiron Machine Learning X Science summer school presents both topics which are at the core of modern Machine Learning, but also topical applications of modern ML on a variety of scientific disciplines. The speakers are leading experts in their field who talk with enthusiasm about their subjects.
The Summer School will consist of two weeks of lectures and 6 weeks of hands-on topics mentored by leaders in the field.
Invited Lecturers:
Noam Brown
Giuseppe Carleo
Kyunghyun Cho
Barbara Engelhardt
Yann Lecun
Internal Lecturers:
Mitya Chklovskii
SueYeon Chung
Miles Cranmer
Robert M. Gower
Shirley Ho
David Hogg
Dan Foreman-Mackey
Stephane Mallat
Chirag Modi
Javier Robledo-Moreno
Vikram K. Mulligan
Anirvan Sengupta
Eero Simoncelli
Alex Williams
Yuling Yao
Frank Zijun Zhang
Wenda Zhou
Due to mentoring capacity limits, we will only be able to admit ~20 applicants to our school in person, but will offer online participation for the first 2 weeks. Please check the box of “online application” if you would also like to be considered for online participation. Our lectures will be streamed online, and we will aim to open source all additional materials.
Eligibility Criteria
– Full time students who are interested in the intersection of Machine Learning and Science. We especially welcome early to mid stage PhD students with a good technical background. Exceptional masters students and undergraduate students who show strong interest or track record in ML X Science will also be considered.
– Postdoctoral fellows who are interested in transitioning into the field of ML X Science from a technical field.
Language: The language of choice throughout the program is English.
Accommodations
Simons Foundation will sponsor admitted participants for the duration of the summer school for their room and board, and travel to and from the summer school.
To begin the application process, please click ‘Apply Now‘.
- Application Deadline
- Program Dates
- to
- Please send all inquiries to
[email protected] - Summer School Organizers:
Miles Cranmer
Domenico Di Sante
Michael Eickenberg
Siavash Golkar
Shirley Ho
Natalie Sauerwald
The Flatiron Machine Learning X Science summer school starts with the motivation to introduce modern methods of statistical machine learning and inference to the scientific community. It was motivated by the observation that while many students are keen to learn about machine learning, and an increasing number of scientists want to apply machine learning methods to their research problems, very few machine learning courses teach state of the art methods with science applications in mind.
Flatiron Machine Learning X Science summer school presents both topics which are at the core of modern Machine Learning, but also topical applications of modern ML on a variety of scientific disciplines. The speakers are leading experts in their field who talk with enthusiasm about their subjects.
Apply here: https://forms.monday.com/forms/0cc00966cbad036601426887bddda5d4?r=use1
Housing in the NYC area will be provided for all participants. Expect to receive additional information if your application is selected
- Application Deadline
- Program Dates
- to
- Please send all inquiries to
[email protected] - Summer School Organizers:
Miles Cranmer
Domenico Di Sante
Michael Eickenberg
Siavash Golkar
Shirley Ho
Natalie Sauerwald
Maria Avdeeva will co-mentor on the following topics:
1. Dimensionality reduction for large-scale tissue flows in developing embryos
2. Representation and modeling of spatiotemporal gene expression datasets
1. Dissecting variance in deep learning: in this topic, a student (or a team of students) chooses one or two benchmark tasks, trains a set of deep neural nets on each of these tasks while varying as many sources of variations as possible (see, e.g., https://kyunghyuncho.me/how-to-think-of-uncertainty-and-calibration-2/) and analyzes the impact of these sources of noise on the final variance of each of different outcome metrics, such as accuracy, entropy, etc.
1. Exploring the connection between the structure in the neural network connectivity (e.g. synaptic weights) and the geometry of neural representations, both in feedforward and recurrent neural network settings.
2. Exploring the efficiency of various bioplausible learning rules from theoretical perspectives (e.g. their match to the backpropagation, and the efficiency of the learning dynamics), with a particular focus on broadcasting global error signals, including but not limited to Global Error Vector Broadcasting (GEVB) rule.
1. Learning new analytic models in astrophysics using symbolic regression
2. Using deep learning to accelerate symbolic regression and model discovery
3. Applications of machine learning to fluid dynamics
1. What physics can we learn about interacting electrons by using artificial intelligence based methods? The research will be conducted within the framework of the NeuralFRG architecture that we have recently developed at CCQ to learn the functional Renormalization Dynamics for correlated fermions (arXiv:2202.13268). The technical part of the research program will also aim at: i) restructuring the code from Python to Julia, in order to efficiently benefit from the Adjoint method for training Neural ODE solvers; ii) extension to other kinds of fRG dynamics; iii) implementation of multi-GPU training.
2. Application of dimensionality reduction algorithms to the ab-initio molecular dynamics for solid state systems. Use of deep-learning architectures to find representations of Koopman eigenfunctions from data and identify coordinate transformations that will make non-linear ab-initio molecular dynamics approximately linear. The aim is to enable nonlinear prediction of these ab-initio dynamics, their extrapolation in future and control using linear theory.
1. Live-cell imaging is an inexpensive way to study the behavior of modified cells. But the methods to fully characterize behavior of cells in these videos do not exist. In this topic, we will use CAR-T live cell imaging videos to motivate the development of machine learning methods that describe the behavior of these modified cells.
2. While single-cell RNA-sequencing has allowed an unprecedented look at transcription within single cells, methods to quantify variation and associate transcription levels with genotypes including expression QTL methods rely heavily on pseudo-bulk methods. In this topic, we will build ML methods for association testing in single cell RNA-seq data that take advantage of the multiple cell read-outs per sample.
1. Developing a framework using analytical tools (NTK, replica theory etc.) to do active learning (finding relevant training samples that maximally improve generalization) and neural architecture search.
2. How does curriculum learning affect adversarial robustness of a neural net? A possible explanation of adversarial susceptibility of neural networks is that they are trained on complicated datasets from scratch. This is akin to taking advanced classes without knowing the prerequisites and learning the wrong lessons.
1. Testing and designing stochastic optimization methods for learning models that almost interpolate.
2. A new natural policy gradient method: Exploiting curvature for RL.
1. Explore astrophysical applications of NLP models.
2. Explore the neural tangent kernel and neural architecture search.
Pearson Miller will co-mentor on the following topics:
1.Dimensionality reduction for large-scale tissue flows in developing embryos
2. Representation and modeling of spatiotemporal gene expression datasets
1. While we often think of genes as functional units of the genome, the same gene can be modified to form multiple isoforms which can vary significantly in their respective functions. This topic will apply machine learning methods to integrate isoform and structural data to better understand the functional implications of different genetic isoforms.
1. Learning dynamics from data: We would like to benchmark different models for learning latent dynamics for scientific problems.
Stanislav Y. Shvartsman will co-mentor on the following topics:
1. Dimensionality reduction for large-scale tissue flows in developing embryos
2. Representation and modeling of spatiotemporal gene expression datasets
1. Application of machine Learning in cosmological challenges
1. This topic will analyze camera and microphone array recordings of Mongolian gerbil families in collaboration with labs run by professors Dan Sanes and David Schneider at NYU. Gerbils are highly social rodents — more so than typical laboratory species — and they produce elaborate vocal call sequences in their social interactions. From these video and audio data, researchers want to extract all vocalizations and identify which gerbil produced them (a problem known as speaker diarization). To achieve this, this topic plan to utilize deep convolutional networks and associated methods to quantify uncertainty in deep learning models.
1. Explainable graph neural network approach for cancer risk predictions
The aim of this topic is to make explainable predication of tumor grade classifications and risks for each tumor patient, leveraging personalized whole-genome scale somatic mutations. State-of-the-art deep learning approaches will be applied to model the coding and non-coding mutation effects, and graph neural networks will be used to integrate variant information across the genome. Finally, researchers will use model explaining techniques to distill biomedical insights that help tumor patient treatments.
2. Whole-genome genetic variation effect interrogation in autism
This topic will employ similar underlying computational strategies encompassing deep convolution neural networks and geometric deep learning and apply them to study autism. Researchers will leverage the latest in-house autism cohort collected by SFARI, the largest autism cohort in the world, and explore the genome-wide de novo mutation effects that influence a range of autism-related measures. These include levels and prognosis of autism, comorbidity and other relevant molecular and phenotypical traits.