Machine Learning at the Flatiron Institute
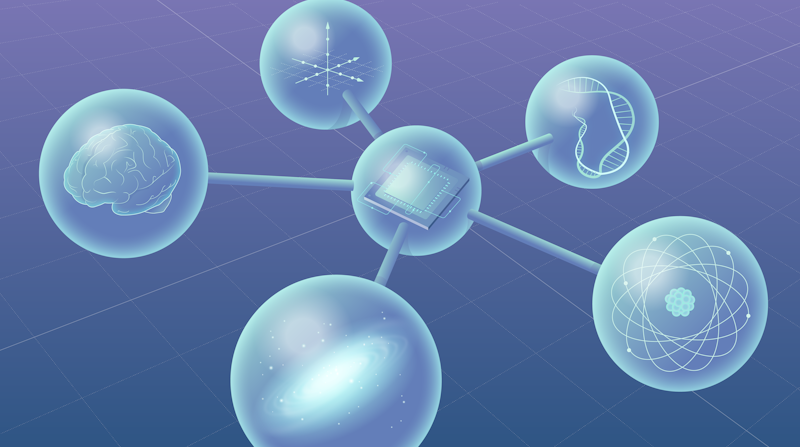
In recent years machine learning has emerged as an indispensable tool for computational science. It is also an active and growing area of study throughout the Flatiron Institute. Researchers at Flatiron are especially interested in the core areas of deep learning, probabilistic modeling, optimization, learning theory and high dimensional data analysis. They are also applying machine learning to problems in cosmological modeling, quantum many-body systems, computational neuroscience and bioinformatics. Below is a list of researchers who work in these areas; prospective visitors should feel free to contact them for more information.
Machine Learning Visiting Scholar Program
Directed by Lawrence Saul (Center for Computational Mathematics) and an advisory committee consisting of Bob Carpenter (CCM), Mitya Chklovskii (Center for Computational Neuroscience), Antoine George (Center for Computational Quantum Physics), David Hogg (Center for Computational Astronomy), Olga Troyanskaya (Center for Computational Biology) and Jutta Rogal (Initiative for Computational Catalysis), the program is designed to spur further collaboration between researchers at the Flatiron Institute and in academia applying machine learning to problems of scientific interest. The program will provide support to current faculty members who wish to spend sabbaticals or leaves of absence at Flatiron and to junior researchers who are able to defer their start date at a tenure-track position in academia in order to spend a research year at the Flatiron Institute.
The program will support up to four visiting scholars at a time, with visits up to one year in length. Junior researchers will be hired as associate research scientists with one-year appointments; senior researchers will typically have a visiting appointment compatible with their university’s sabbatical leave policies. Visiting scholars will be offered subsidized housing, salary and benefits as appropriate, travel and other research-related expenses.
Researchers in CCM
Alberto Bietti
Research Scientist, CCM
Areas of Interest: Learning theory, optimization, deep learning, kernel methods
David Blei
Visiting Scholar, CCM
Areas of Interest: Topic models, probabilistic modeling, approximate Bayesian inference
Joan Bruna
Visiting Scholar, CCM
Areas of Interest: Learning theory, deep learning, machine learning for science, high dimensional statistics, algorithms
Andreas Buja
Senior Research Scientist, CCM
Areas of Interest: Statistical methodology, model misspecification, replicability, causality, applications in the genetics of autism
Diana Cai
Flatiron Research Fellow, CCM
Areas of Interest: Probabilistic modeling, robust Bayesian inference, machine learning for science
Bob Carpenter
Senior Research Scientist, CCM
Areas of Interest: Probabilistic programming, Markov chain Monte Carlo methods, variational inference
Michael Eickenberg
Research Scientist, CCM
Areas of Interest: Machine learning for science, applied statistics and signal processing, deep learning, neuroimaging and computational cognitive neuroscience
Anna Gilbert
Visiting Scholar, CCM
Areas of Interest: Theory and algorithms for high dimensional data analysis, metric representations, non-Euclidean embeddings
Robert Gower
Research Scientist, CCM
Areas of Interest: Stochastic optimization, interpolation, adaptive methods for deep learning, convergence of algorithms and second order methods
Jiequn Han
Flatiron Research Fellow, CCM
Areas of Interest: Multiscale modeling, numerical methods for partial differential equations, machine learning for science
Stephane Mallat
Distinguished Research Scientist, CCM
Areas of Interest: Signal processing, harmonic analysis, deep learning
Charles Margossian
Flatiron Research Fellow, CCM
Areas of Interest: Probabilistic programming, Markov chain Monte Carlo methods, variational inference
Rudy Morel
Flatiron Research Fellow, CCM
Areas of Interest: Machine learning for science, deep learning, high-dimensional statistics, signal processing
Ruben Ohana
Flatiron Research Fellow, CCM
Areas of Interest: Deep learning, randomized algorithms, high dimensional statistics, differential privacy
Loucas Pillaud-Viven
Flatiron Research Fellow, CCM
Areas of Interest: Learning theory, optimization, deep learning
Bruno Régaldo-Saint Blancard
Flatiron Research Fellow, CCM
Areas of Interest: Machine learning for astrophysics, applied signal processing, generative modeling
Neha Wadia
Flatiron Research Fellow, CCM
Areas of Interest: Learning theory, continuous-time optimization, high dimensional statistics
Lawrence Saul
Group Leader, Machine Learning, CCM
Areas of Interest: High dimensional data analysis, latent variable models, deep learning, variational inference, kernel methods
Berfin Şimşek
Guest Researcher, CCM
Areas of Interest: Learning theory, loss landscape analysis, deep Learning
Yuling Yao
Flatiron Research Fellow, CCM
Areas of Interest: Scalable Bayesian workflows, meta-learning, causal inference
Researchers in CCN
Mitya Chklovskii
Group Leader, Neural Circuits and Algorithms, CCN
Areas of Interest: Theoretical neuroscience, connectomics, biologically inspired AI, dynamics and control
SueYeon Chung
Project Leader, Geometric Data Analysis, CCN
Areas of Interest: Theoretical neuroscience, statistical physics of learning, high dimensional geometry and statistics
Jenelle Feather
Flatiron Research Fellow, CCN
Areas of Interest: Theoretical neuroscience, analysis of high dimensional auditory and visual representations
Siavash Golkar
Associate Research Scientist, Neural Circuits and Algorithms, CCN
Areas of Interest: Biological learning, deep learning, machine learning for science
Sarah Harvey
Flatiron Research Fellow, CCN
Area of Interest: Theoretical neuroscience, statistical physics, ML methods for neural data analysis
Brett Larsen
Flatiron Research Fellow, CCN/CCM
Areas of Interest: Deep learning, optimization, loss-landscape analysis, sparsity, high-dimensional statistics
David Lipshutz
Associate Research Scientist, CCN
Areas of Interest: Theoretical neuroscience, neuro-inspired ML, stochastic analysis, dynamical systems
Amin Nejatbakhsh
Flatiron Research Fellow, CCN
Areas of Interest: Computational neuroscience, machine learning, statistics, dynamical systems, computer vision
Eero Simoncelli
Director, CCN
Areas of Interest: Analysis and representation of visual information in biological and artificial networks. Coding and inference
Tiberiu Tesileanu
Associate Research Scientist, Neural Circuits and Algorithms, CCN
Areas of Interest: Biological learning, deep learning
Alex Williams
Associate Research Scientist, Statistical Analysis of Neural Data, CCN
Areas of Interest: Unsupervised learning, uncertainty quantification in deep learning, topological data analysis, covariance estimation
Researchers in CCQ
Anna Dawid
Flatiron Research Fellow, CCQ
Areas of Interest: Machine learning for (quantum) science, interpretability, deep learning theory
Domenico Di Sante
Affiliate Research Fellow, CCQ
Areas of Interest: Theoretical neuroscience, statistical physics of learning, high dimensional geometry and statistics
Antoine Georges
Director, CCQ
Areas of Interest: Machine learning for quantum systems
Matija Medvidović
Graduate Student, CCQ
Areas of Interest: Machine learning for many-body quantum physics, sampling, optimization
Andrew Millis
Co-Director, CCQ
Areas of Interest: Theoretical condensed matter physics, high-temperature superconductivity, numerical methods for the many-electron problem
Alev Orfi
Graduate Student, CCQ
Areas of Interest: Neural quantum states, variational Monte Carlo, sampling algorithms
Anirvan Sengupta
Visiting Scholar, CCQ
Areas of Interest: Representation learning, dynamics and control, applications to quantum systems, systems neuroscience
Christopher Roth
Flatiron Research Fellow, CCQ
Areas of Interest: Neural quantum states, equivariant models, variational Monte Carlo
Jaylyn C. Umana
Graduate Student, CCQ
Areas of Interest: Symbolic regression, neural networks, optimization
Agnes Valenti
Flatiron Research Fellow, CCQ
Areas of Interest: TBD
Jiawei Zang
Graduate Student, CCQ
Areas of Interest: Machine learning for many-body quantum physics, dimensionality reduction
Researchers in CCB
Xi Chen
Research Scientist, CCB
Areas of Interest: Distribution learning, Markov chain Monte Carlo, semi-supervised learning
Adam Lamson
Flatiron Research Fellow, CCB
Areas of Interest: Interpretable neural networks, biophysical and genomics modeling, reservoir computing
Suryanarayana Maddu
Flatiron Research Fellow, CCB
Areas of Interest: Physics-informed machine learning, statistical learning theory, high dimensional statistics
Zhicheng Pan
Flatiron Research Fellow, CCB
Areas of Interest: Deep learning for genomics, graphical neural networks
Christopher Park
Research Scientist, CCB
Areas of Interest: Probabilistic modeling, deep learning and statistical genetics
Natalie Sauerwald
Flatiron Research Fellow, CCB
Areas of Interest: Machine learning for genomics and genetics, optimization, interpretable models
Rachel Sealfon
Research Scientist, CCB
Areas of Interest: Machine learning for genomics, analysis of functional genomic data
Olga Troyanskaya
Deputy Director for Genomics, CCB
Areas of interest: Genomics and bioinformatics
Mao Weiguang
Flatiron Research Fellow, CCB
Areas of Interest: Deep learning, graphical models, dimensionality reduction
Researchers in CCA
Miles Cranmer
Flatiron Research Fellow, CCA
Areas of Interest: Open-source tooling, model interpretability, learning to simulate, learned coarsening, physics-based inductive biases, graph neural networks, sparsity, symbolic regression/program synthesis, model distillation
Daniel Foreman-Mackey
Research Scientist, CCA
Areas of Interest: Probabilistic programming, Markov chain Monte Carlo, Gaussian Processes
Shirley Ho
Group Leader, Cosmology X Data Science, CCA
Areas of Interest: Machine learning for science, deep learning for simulation, neuro-symbolic models, high dimensional inference
David W. Hogg
Group Leader, Astronomical Data, CCA
Areas of Interest: Causal models, enforcing physical symmetries, adversarial attacks, models of cameras and spectrographs
Chirag Modi
Flatiron Research Fellow, Cosmology X Data Science, CCA joint with CCM
Areas of Interest: Machine learning for science, differentiable simulations, Markov chain Monte Carlo methods, approximate Bayesian inference
Francisco Villaescusa-Navarro
Research Scientist, CCA
Areas of Interest: Neuro-simulations, graph neural netwoks, likelihood-free inference, manifold learning, generative models, symmetries for deep learning.
Kaze Wong
Flatiron Research Fellow, Gravitational Wave Astronomy, CCA
Areas of Interest: Deep learning for data analysis and simulation in astrophysics
Events
Machine learning events at Flatiron Institute come in two flavors: one-time events like workshops, conferences or schools and a regular seminar series, ML@FI. You can find incoming events and selected archived past events below.
ML@FI is a seminar series focused on machine learning and its applications to science. It is aimed at Flatiron Institute research scientists and our collaborators. Seminars usually take place every other Tuesday at 3:00 p.m. in the CCN classroom on the fourth floor of 160 Fifth Ave or in the CCA 5th floor classroom at 162. Each meeting is followed by a reception to encourage inter-center interactions. See the website for past seminars and their recordings!
For more information, to join the seminar mailing list or to propose speakers for future seminars, please contact the organizers: Shirley Ho, Alberto Bietti, and Francois Lanusse.
The Machine Learning New York City (ML-NYC) Speaker Series is a monthly event for machine learning practitioners, researchers, and students to meet and watch talks from leading researchers in the field. Each event will feature a New York City-based speaker presenting their work. The ML-NYC Speaker Series is open to anyone interested in machine learning, and we encourage everyone to attend, whether you are a beginner or an expert in the field.
For more information or to propose speakers for future seminars, please contact the organizers: Lawrence Saul, David Blei and Joan Bruna
-
01 Tue -
Seminar 4:00 - 6:00 p.m.
Machine Learning at the Flatiron Institute: Clément Hongler
-
Seminar 4:00 - 6:00 p.m.
-
08 Tue -
Lecture 4:00 - 6:00 p.m.
Machine Learning at the Flatiron Institute: Danqui Chen
-
Lecture 4:00 - 6:00 p.m.
-
17 Thu -
Lecture 4:00 - 5:00 p.m.
Flatiron Seminar Series: Matteo Cantiello
-
Lecture 4:00 - 5:00 p.m.
-
29 Tue -
Seminar 4:00 - 6:00 p.m.
Machine Learning at the Flatiron Institute: Akari Asai
-
Seminar 4:00 - 6:00 p.m.
-
01 Thu -
Seminar 3:00 - 4:00 p.m.
ML@FI Seminar Series
-
Seminar 3:00 - 4:00 p.m.
-
01 Sun -
Seminar 3:00 - 4:00 p.m.
ML@FI Seminar Series
-
Seminar 3:00 - 4:00 p.m.
-
01 Tue -
Seminar 3:00 - 4:00 p.m.
ML@FI Seminar Series
-
Seminar 3:00 - 4:00 p.m.
-
01 Fri -
Seminar 3:00 - 4:00 p.m.
ML@FI Seminar Series
-
Seminar 3:00 - 4:00 p.m.
-
01 Mon -
Seminar 3:00 - 4:00 p.m.
ML@FI Seminar Series
-
Seminar 3:00 - 4:00 p.m.
-
01 Wed -
Seminar 3:00 - 4:00 p.m.
ML@FI Seminar Series
-
Seminar 3:00 - 4:00 p.m.
Past Events
Cosmic Connections: A ML X Astrophysics Symposium
Flatiron-Wide Machine Learning (FWML)
2022 Machine Learning at the Flatiron Institute Seminar Series
2021 Machine Learning at the Flatiron Institute Seminar Series
2020 Machine Learning at the Flatiron Institute Seminar Series
Flatiron Machine Learning X Science Summer School
Challenges and Prospects of Machine Learning for the Physical Sciences
Learn the Universe — an ML X Cosmology Workshop
Machine Learning for Quantum Simulation: Virtual Conference
Machine Learning Quantum Matter Data