Foundation Models of Science
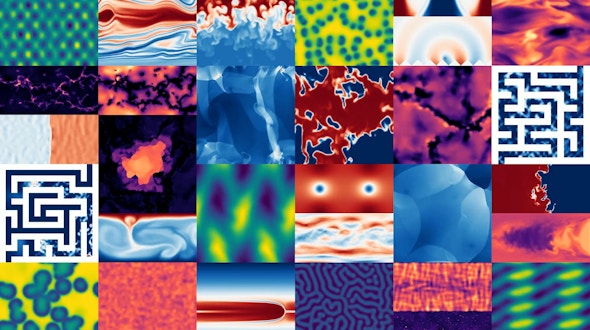
Our goal is to accelerate the development of versatile foundation models tailored for numerical datasets and scientific machine learning tasks. The challenge we are undertaking is to build AI models which leverage information from heterogeneous datasets and across different scientific fields, which, contrary to domains like natural language processing, do not share a unifying representation.
Such models can then be used as strong baselines or be further fine-tuned by scientists for specific applications. This approach has the potential to democratize AI in science by providing off-the-shelf models that have stronger priors for shared general concepts such as causality, measurement, signal processing, and even more specialized shared concepts like wave-like behavior, which otherwise would need to be learned from scratch.
Researchers
Alberto Bietti
Flatiron Institute
Center for Computational Mathematics
Kyunghyun Cho
New York University
Miles Cranmer
Data Intensive Science
University of Cambridge
Michael Eickenberg
Flatiron Institute
Center for Computational Mathematics
Shirley Ho
Flatiron Institute
Center for Computational Mathematics
Keiya Hirashima
University of Tokyo
Tom Hehir
Institute of Astronomy
University of Cambridge
Siavash Golkar
New York University
Geraud Krawezik
Flatiron Institute
Scientific Computing Core
Francois Lanusse
Flatiron Institute
Center for Computational Astrophysics
Nick Lourie
New York University
Michael McCabe
University of Colorado
Ruben Ohana
Flatiron Institute
Center for Computational Mathematics
Payel Mukhopadhyay
University of California, Berkeley
Rudy Morel
Flatiron Institute
Center for Computational Mathematics
Lucas Meyer
Polymathic AI
Liam Parker
Flatiron Institute
Center for Computational Astrophysics
Mariel Pettee
Lawrence Berkeley National Laboratory
Bruno Regaldo
Flatiron Institute
Center for Computational Mathematics
Scientific Advisory Group
Colm-Cille Caulfield
University of Cambridge
Leslie Greengard
Flatiron Institute
New York University
David Ha
Sakana AI
Yann LeCun
Meta AI
New York University
Stephane Mallat
École Normale Supérieure
Collège de France
Flatiron Institute
David Spergel
Simons Foundation
Olga Troyanskaya
Flatiron Institute
Princeton University
Laure Zanna
New York University