Machine Learning X Astrophysics
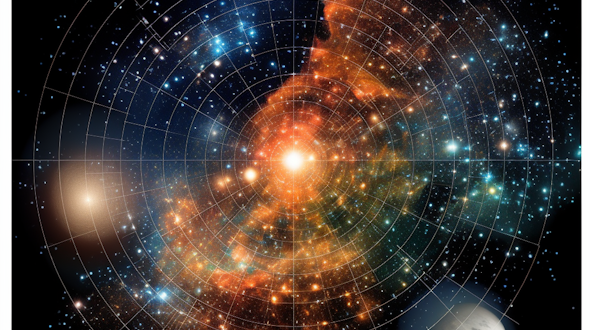
Machine learning is being increasingly integrated into scientific discovery to augment and accelerate research, helping scientists to generate hypotheses, design experiments, collect and interpret large datasets, and gain insights that might not have been possible using traditional scientific methods alone. Researchers at CCA is leading the wave of rapid development and adoption of machine learning techniques to enable and accelerate scientific discovery.
In particular, researchers at CCA led the first application of 3D convolutional neural network in astrophysics, first application of machine learning to accelerate cosmological simulations, first development of deep learning accelerated simulation based inference for large scale spectroscopic surveys and first combined symbolic regression with neural network to extract scientific insights. More recently, researchers at CCA and their collaborators are leading the development of Foundation Model for Sciences and are excited to discover what large pre-trained models can do for science in general.
Subgroup
Polymathic AI
Polymathic AI researches a new class of foundation models for scientific data, developing models that share scientific concepts across disciplines. The Polymathic AI initiative aims to accelerate the development of versatile foundation models tailored for machine learning tasks in science. A breakthrough in this field would mark a significant shift analogous to the one seen in vision and natural language processing (NLP). The team comprises both pure machine learning researchers and domain scientists, covering a range of disciplines. A scientific advisory group of world-leading experts guides Polymathic AI.
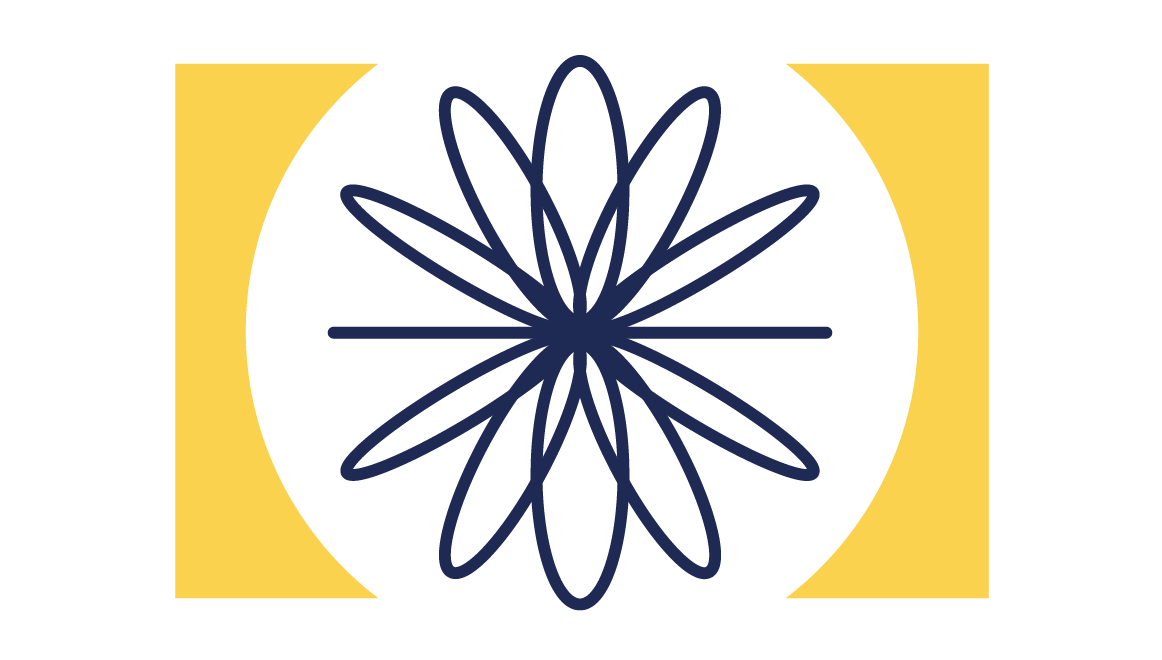