A New Benchmark to Identify the Best Solutions to the Hardest Problems in Materials Science
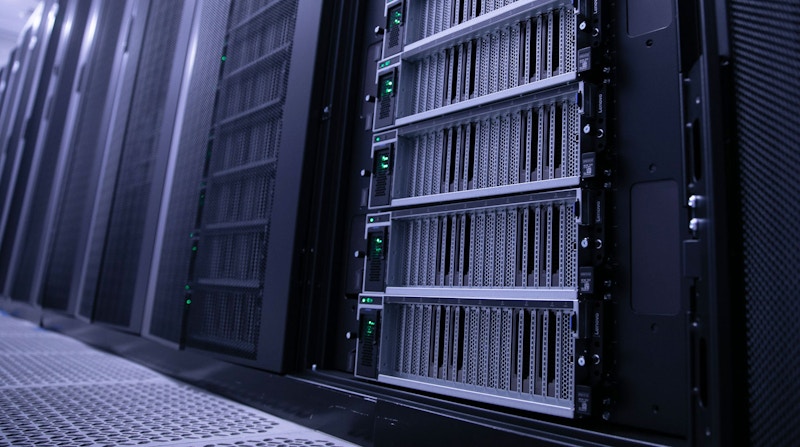
Quantum computing — technology that leverages quantum mechanics to solve problems differently than classical computers — holds a lot of promise for helping scientists understand complex materials. However, there hasn’t been an easy way for researchers to determine the cases in which quantum methods will outperform their classical counterparts in simulating such materials.
New research establishes a test called a V-score that can compare the performance of the computers’ different algorithms to determine the best option for understanding different materials. This work was led by Giuseppe Carleo of the École Polytechnique Fédérale de Lausanne (EPFL) and the National Centres of Competence in Research (NCCR) MARVEL in Switzerland and carried out in collaboration with scientists at the Flatiron Institute Center for Computational Quantum Physics (CCQ). The study appears in the October 18 issue of Science.
“Here at CCQ, we develop methods and algorithms to compute the properties of large interacting quantum systems like those described in this study,” says CCQ Director Antoine Georges. “We are excited that our state-of-the-art methods were able to help establish this crucial tool that will help streamline future research into quantum systems by providing a reliable benchmark of how accurately different algorithms approximate them.”
Matchmaking for Problem Solving
The study focuses on quantum many-body problems, in which scientists try to predict the behavior of quantum particles interacting with each other. When many of these particles are in play, this can become extraordinarily difficult.
For example, one challenge in physics is to calculate the ground state of a material, or the lowest possible energy level at which the material can exist. The ground state is also used to determine whether it could become a superconductor — an important class of material used in everything from electric motors to MRIs. This is a many-body problem that is typically approximated with classical algorithms but that might be best solved by using a quantum system. However, there hasn’t been an established tool that can determine whether a quantum system will outperform the classical algorithms at solving this kind of problem — until now.
The new V-score (‘V’ for ‘variational accuracy’) uses two pieces of information — the energy in a system and how that energy fluctuates — to determine which type of algorithm can best solve a problem. The higher the V-score, the harder a problem is to solve with a traditional algorithm, pointing to places where quantum computing could make the biggest impact.
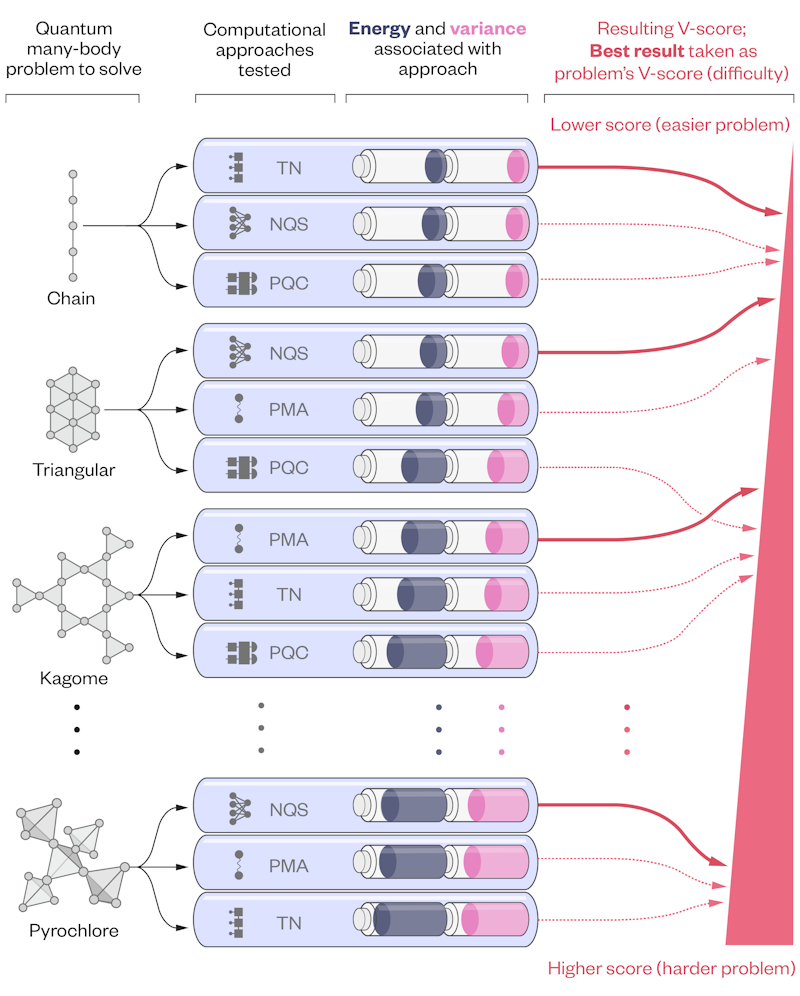
So, for one-dimensional materials like carbon nanotubes, a classical method will probably get the job done, producing lower V-scores in the process. For more complex materials such as three-dimensional crystal structures where particles have fewer predictable interactions due to their magnetic properties, a high V-score suggests an area where a quantum system could outperform.
“This does not mean [low V-score problems] are simple per se, but that we have good techniques to tackle them,” Carleo says.
A Trustworthy Toolbox
For Georges, one exciting outcome of this work is that it provides benchmarks for future demonstrations of ‘quantum advantage’ (the notion that quantum systems will always work better than a classical one) while demonstrating that implementations of classical algorithms currently outperform their quantum counterparts.
“What’s most exciting to me is that our work demonstrates that algorithms operating on classical computers still outperform the capabilities of quantum computers,” says Georges. “So, future works aiming at establishing ‘quantum advantage’ on a specific problem will have to demonstrate that it can outperform the V-score established in our paper for that problem first.”
This tool is now available to the wider scientific community to accelerate materials science research and determine how advantageous new techniques will be at solving problems. This will cut down on the time and money typically spent to make this assessment.
“Our database and codes are fully open access, and we’d like it to become a dynamic resource that is updated whenever a new technique is introduced in the literature,” Carleo says.
It will also help inform the development of these new techniques.
“For Flatiron, one exciting outcome of this work is that it provides a way to identify difficult problems that will challenge our algorithms and drive the next stages in our method development and application,” says CCQ Co-Director Andrew Millis. “The study has already identified a few of these problems, and we’re looking forward to what it will enable in the future.”
For more information, please contact Stacey Greenebaum at press@simonsfoundation.org.