Tapping into the Body’s Natural Electricity to Improve Treatments for Neurological Disorders
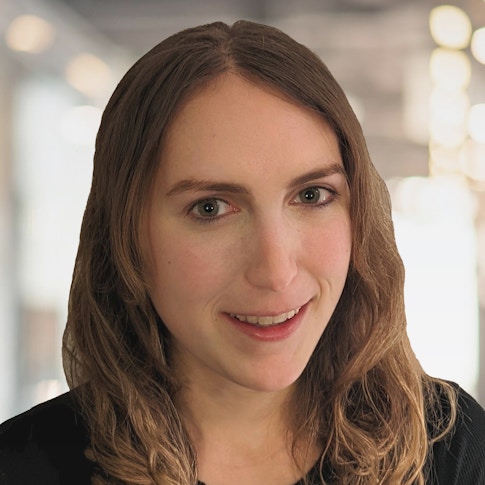
When she entered college, Cynthia Steinhardt was already interested in biology. An elective introductory neuroscience course soon sparked her fascination with the brain and its unparalleled computational abilities.
Today, Steinhardt is a postdoctoral research fellow working with Larry Abbott in Columbia University’s Center for Theoretical Neuroscience, where she has just completed her first year as a Junior Fellow with the Simons Society of Fellows. At Columbia, she combines her knowledge of brain-computer interfaces with the latest in theoretical neuroscience to understand how the brain uses electrical signaling to communicate — and how that knowledge could lead to improved treatments for neurological disorders.
Prior to joining Columbia, Steinhardt earned a doctorate in biomedical engineering, with a focus in neuroengineering, from the Johns Hopkins School of Medicine. She holds a bachelor’s degree in neuroscience, with a concentration in cognitive science, from Princeton University.
Steinhardt and I recently discussed her scientific path and current work. Our conversation has been edited for clarity.
What drew you to your line of research?
Initially, I was excited to learn how the brain acts like a biological computer — how it helps us to do the complex tasks that make us innately human: to speak languages, create art or feel emotions. When I took my first neuroscience course, I was surprised to discover that even seemingly simple brain tasks — such as remembering an image or basic motor skills — are complex too. They require a precise choreography of activity involving different neural populations across disparate brain regions. And while there is still a lot to learn about even the most studied aspects of the brain, one thing we do know is that the brain has evolved optimal solutions for storing information and computing.
Theoretical neuroscience has helped us to advance our understanding of these abilities. It enables us to better understand the biology of the brain and think about its functions on an algorithmic level using the language of math.
In college, I became drawn to theoretical neuroscience. At the same time, I was considering becoming a doctor so that I could work directly with patients suffering from mental illness and neurological diseases.
My path forward became clear during a class at Princeton, when I saw a video of a man who was quadriplegic using a brain-computer interface to help him interact with his surroundings. With electrodes implanted in his brain, the system could analyze neural activity in the man’s motor cortex, decode his intended motions, and tell a robotic arm to pick up a glass of water for him to drink. Seeing this helped guide me toward an area of research that was a perfect fit for all my interests: biomedical engineering. In this field, I could study the complexity of the healthy and impaired brain and use that understanding to help design treatments that could restore neural function. This realization led me to pursue a Ph.D. in biomedical engineering at the Johns Hopkins School of Medicine, where I studied state-of-the art neural implants and interfaces.
How can theoretical/computational work help to improve neural implants in patients?
While an important aspect of designing neural implants is making the hardware biocompatible and efficient for delivering the right currents to the brain, it is equally important to ask what type of signals the brain needs to receive to restore function. Current technologies limit the level to which we can probe the brain and the types of questions we can ask experimentally, so this is part of where computational research can help.
Computational work allows us to simulate what we hypothesize to be the important features of an experiment and the biology of the brain, as well as to investigate features of our simulations in ways we cannot yet experimentally. We can also use the information we learn from that process to design new algorithms for neural implants that incorporate this understanding of electricity and the brain.
How did you employ this approach at Johns Hopkins?
I originally came to Hopkins to understand neural interfaces: devices implanted in the body that interact with the brain. I took a course taught by Gene Fridman that detailed the engineering and biology behind neural implants used to treat a variety of diseases — from Parkinson’s disease to hearing loss to chronic pain. Professor Fridman eventually became my Ph.D. advisor, and with him I began to ask questions about how we could improve neural implants.
Neural implants rely on electrical stimulation to activate and deactivate neural populations involved in a particular pathology. My Ph.D. work focused on exactly what electrical stimulation does to targeted neurons and whether we could improve those interactions to better control local populations of neurons.
Specifically, we learned how short bursts of current, or pulses, affect neurons. All neural implants use pulses to drive brain activity, and most algorithms assume a linear relationship between pulse rate and neural activity (or pulse amplitude and neural activity). To increase neural activity, we increase the pulse parameters.
But I found that relationship to be more complicated. Pulses are highly unnatural; they can both facilitate and block the brain’s natural neural activity. They can also both facilitate and block other pulses from modulating neural activity. These effects depend on the pulse parameters and the neurons’ natural activity, meaning that even the exact same sequence of pulses might activate one neuron while simultaneously blocking another. Part of my work asked how we could program neural interfaces to deliver currents that produce more accurate neural firing patterns.
This led me to my current research on cochlear implants: small electrical devices that mimic the function of the inner ear — the cochlea — for people who are profoundly deaf. Broadly, these implants take sound from one’s environment, process it, and use electrical currents to activate spiral ganglion cells that send information to the rest of the brain’s auditory system. Based on my Ph.D. results, we expect the spiral ganglion cells of people with cochlear implants to produce signals differently than those of hearing individuals. But the brains of those with cochlear implants are still somehow extracting a similar enough signal to enable hearing capabilities. At Columbia, I’m working to shed some light on the mechanisms behind this process.
How are you doing that?
The cochlea contains thousands of neurons that break down sound into 20,000 distinct frequencies. Cochlear implants use 20–30 electrodes, compressing sounds into only eight frequencies. Even with just eight frequencies, people with cochlear implants understand speech in real time — something that our most advanced machine learning algorithms still struggle to do. Despite this technological feat, wearers struggle to distinguish tones. My research at Columbia asks why cochlear implants are good at restoring speech perception, while being much less adept at imparting tonal information.
I’m making simulations of how the cochlea naturally encodes information, and I’m training advanced computer models called neural networks to respond to that information. The models are trained to perform the same assessments that would be performed in a clinic with normal-hearing individuals. I also simulate how the implants activate the cochlea and analyze how the neural activity throughout the network differs. Understanding this difference could, I hope, lead to improvements in the way cochlear implants function. I’m currently collaborating with experimental neuroscientists at New York University (NYU) who are studying how neural populations in the rat brain respond to cochlear implant stimulation and how their brains adapt to use it better over time. Through this collaboration, I hope to validate my models, testing new theories for how we can drive networks of neurons performing a complex task with electrical stimulation.
Finally, what are your thoughts about the Simons Junior Fellowship?
It is only my first year, but it has already been a pleasure to be part of this community. During my postdoc, I changed fields from engineering to basic science. The mentorship from Senior Fellows helped make that transition almost seamless. The connections helped me lay the groundwork for building my career and even helped me to find ideal collaborators at NYU.
I’ve chosen a unique problem that requires theoretical, computational, engineering and experimental work. Having the Simons Foundation’s support has given me the time and freedom to find the right way to pursue my research questions and build new collaborations across institutions.
Finally, the friendships I have developed with other Junior Fellows have been invaluable. Our conversations together, as well as those with visiting lecturers, have taught me how to convey the core of why I do the work that I do and to see the beauty of problems in very different fields. I feel truly lucky to have had this opportunity and look forward to the next two years.