
Neuroscientists Grapple With New Data Management Rules
This month marks a new era in how federally funded scientists think about their data. Beginning on January 25, researchers applying for grants from the National Institutes of Health must create a specific plan for how they will manage and store their data. The far-reaching new policy aims to boost the progress of science by improving reproducibility and encouraging more extensive use of data that is often expensive to collect. But it has also sparked concern over the time and effort required to meaningfully share data.
“I think the pandemic and research on COVID illustrated how much faster we can communicate and move science forward if we make data available,” says Lyn Jakeman, director of the division of neuroscience at the National Institute of Neurological Disorders and Stroke. Jakeman notes that planning for the new policy, first announced in 2020, began long before the pandemic.
The new regulations, set in motion a decade ago by a federal dictate to make publicly funded research more broadly available, arrive amid a growing push for data-sharing across scientific fields. Many scientific journals mandate or encourage data-sharing, as do non-federal grant agencies. The NIH already requires sharing of clinical and genomic data, as well as data generated from large-scale projects. But the new policy aims to tame a very different beast — the data produced from a wide range of basic science fields. Basic science involves experimental trial and error and methodological variability, both of which make data-sharing much more difficult.

Sharing and standardizing data has been a notorious challenge for neuroscience, particularly systems neuroscience, because of the diversity of data involved. Unlike, say, genomics or brain imaging, which typically uses standardized instruments, neurophysiology often employs custom-built tools and bespoke data processing pipelines, which produce data in a range of formats that can be difficult to share across labs. Moreover, the information that accompanies neurophysiology experiments, known as metadata, such as the animal’s behavior, genetics and other factors, can be difficult to keep straight. “Correlated data can be really hard to manage because you have to make sure connections across files and data types are maintained,” says Maryann Martone, a neuroscientist at the University of California, San Diego, who has been heavily involved in open science efforts. (For more on the challenges of data-sharing and standardization in neuroscience, see our 2019 series “The Data-Sharing Problem in Neuroscience.”)
A number of new tools for standardizing, storing and processing neuroscience data have been developed over the last five years, but their adoption is far from comprehensive. While most researchers recognize the broad benefits of making data more easily and broadly available, the best way to do so remains a point of debate. Some want to settle on standardized tools, while others want to continue to experiment, and both ends of the spectrum require more buy-in from the community to be effective. Funders and others hope that the combination of new tools and the federal mandate will help catalyze more widespread use. “I’m a fan of the requirement even though we’re kind of not ready for it,” says Stephen Van Hooser, a neuroscientist at Brandeis University. “I think the typical lab will initially not do very well, but the requirements will stimulate development of new and better approaches to making data-sharing easier.”
“I think we are at a tipping point; that’s what this policy reflects,” says Satrajit Ghosh, a principal research scientist at the Massachusetts Institute of Technology who co-leads an NIH-funded repository of neurophysiology and cellular imaging data. “With the growth of public clouds, as well as improvements in research computing infrastructure, we now have the capacity to store and process large-scale data.”
For systems neuroscience and neurophysiology data specifically, available tools include Neurodata Without Borders (NWB), a platform for standardizing how neuroscience data are stored, and the Distributed Archives for Neurophysiology Data Integration (DANDI), a platform for publishing, sharing and processing neurophysiology data.
Reactions to the new policy run the gamut from apprehension to a sort of reluctant gratitude. Many researchers are concerned about the time and resources required to follow the new rules. Others say the policy doesn’t go far enough to ensure a meaningful level of data-sharing. Still others appreciate the push to put data-sharing into practice. “I like that this provides an impetus to adopt standardizing practices in our field,” says James Heys, a neuroscientist at the University of Utah and a former fellow with the Simons Collaboration on the Global Brain. “I say this as someone who has not yet done this in my lab but knows it’s the best thing to do in the long run, and as someone who needs an external pressure like this to bring data standardization to the top of the long daily to-do list.”
A culture shift
A central component of the new policy is that investigators must outline at the outset of a project how they will manage and store their data. “This is about having a plan; before you start the experiment, where are you going to put the different kinds of data you’re going to create?” Jakeman says. This requires a shift in thinking for many investigators, she says, herself included. “This is the beginning of developing the mindset that data is as valuable as the story we tell about it in a journal article.”
In contrast to the policies of some institutes or grants, the new NIH-wide requirements do not specify exactly what type of data must be shared, where it must be stored or in what format. Instead, researchers must share data “of sufficient quality to validate and replicate research findings.” The broad nature of the new policy reflects the unsettled landscape of data-sharing across many fields of science. “We want to encourage research communities to build data standards and repositories for their communities that work best for them,” Jakeman says. “Someone doing a clinical study versus nematode neurophysiology will be very different in how they share that data.”
That lack of specificity has raised some questions, such as what level of data — from raw to processed — must be shared. The answer will likely vary across institutes within the NIH, Jakeman says. “There is no one-size-fits-all on whether raw data should be shared.” NIH program staff will review data-sharing plans after peer reviewers have assessed the application’s scientific value. “It will be a distributed NIH staff decision about what granularity of data needs to be shared,” she says. Individual institutes are also grappling with how to define the most valuable types of data. “We can’t define usable at this point for every type of data that is generated,” Jakeman says. “We have to rely on the community to define what is usable, and over time, hopefully, the quality of that data will improve.”
Some researchers are concerned about how meaningfully data will be shared — whether others will be able to understand and analyze it. “At worst, researchers can simply check a box to show they are complying with the requirement but not making their data available in a useful way,” says David Markowitz, a former program manager at the Intelligence Advanced Research Projects Activity (IARPA) who managed a large neuroscience project. “Just making the data available is insufficient to guarantee it’s used for science.” Others like the fact that the new policy does not dictate exactly what types of data must be shared or how, because it enables a period of experimentation in which different fields can figure out the most effective approaches. “I like that they’re not prescribing one solution, that they’re letting the community explore,” Van Hooser says. “I think that’s appropriate, particularly for neurophysiology and optophysiology, where it’s a lot harder to describe and agree on an interpretation than for genomics and other data.”
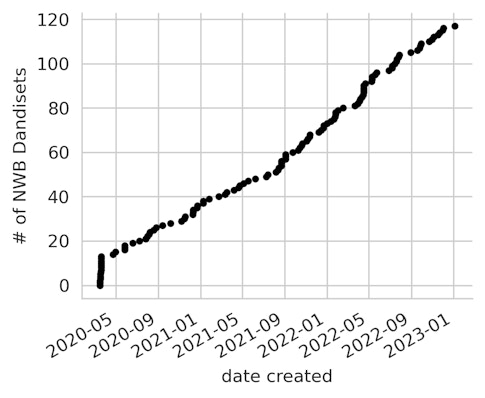
Archives and standards
The BRAIN Initiative has already begun tackling the complexity of data-sharing in neuroscience. Beginning in March 2020, recipients of BRAIN Initiative grants were expected to share the data they collected, and to outline the data standard and archive they planned to use. “That’s a very different and distinct requirement than what the new NIH policy lays out,” Ghosh says. To prepare for the mandate, the BRAIN Initiative funded development of data archives, including DANDI, that are specialized for different types of neuroscience data. Use of the archives is still new, so what people will post and whether the data and metadata are sufficiently annotated to provide lasting value is still an open question.
The NIH-wide data management rules, in contrast, do not specify a particular archive or format that applicants must use, but researchers do need to describe the types of data they will collect, applicable data standards and the repository where data will be stored. Common repositories include Figshare and Mendeley. For neurophysiology data specifically, DANDI and NWB are two NIH-funded candidates. (The SCGB has also helped support NWB.) DANDI currently stores 345 terabytes of data, encompassing more than 100 so-called Dandisets, including a significant number of microscopy datasets.
Cost and capacity are major concerns with large-scale data-sharing, particularly in the long term. DANDI provides scientists with free storage and access, underwritten by the Amazon Web Services public data-sharing program. According to Ghosh, data storage capacity is sufficient for the moment. “We are not scratching our limit at present, so I don’t lose sleep over that right now.” But he says that may change as newer instrumentation generates significantly larger amounts of data. “The community will need to have conversations around data storage and longevity over the next 10 years,” he says. He notes that DANDI has backup storage plans, should issues with Amazon services arise.
Data to be uploaded to DANDI must be in the NWB format, a commonly used standard for neurophysiology data. Interest in NWB has grown since the announcement of the BRAIN and NIH data-sharing mandates, but adoption is still far from widespread. “Right now, conversion is the single biggest challenge for most researchers,” Ghosh says. “Even though it’s not perfect, NWB provides a flexible system that can adapt to changing technologies, and there is an ecosystem of tools growing around it.”
To help new users, NWB and DANDI offer tutorials, documentation and user training workshops. They are also developing new tools, like NeuroConv, an open-source data conversion library that can now handle 36 different common data formats, and an interactive interface, which Ghosh likens to tax submission software, to help researchers convert their data. Ghosh says his team has been developing Jupyter notebooks and other instructional resources for how to use NWB to process and analyze data, aimed at researchers new to NWB and the NIH policy. Though converting data to NWB can be time-consuming, it does have payoffs for labs. Data stored in a broadly readable format is less likely to get lost when lab members leave and makes collaborations easier to develop. “There are incentives,” Ghosh says. “It’s not just distribution; you can do analytics and processing for papers and research.”
In October, NWB held a NeuroDataReHack event, a collaboration with DANDI, the Allen Institute and the Kavli Foundation, to help encourage reuse of existing DANDI datasets. “That’s where data-sharing needs to go — data that’s deposited can be used for reproducibility and to generate new scientific insights,” Ghosh says. “We’re just at the start of that process, I think.”
Others are working on alternative approaches, such as browser-based interfaces and APIs (applied programming interfaces) for working with data. For the MICrONS project, a large-scale collaboration encompassing both anatomical and neurophysiology data that involved petabytes of data, researchers developed a website and API for scientists to explore the data. Markowitz says the NIH has already funded a follow-on project to continue to improve the resource, and researchers are applying for funding to analyze MICrONS data in novel ways. Van Hooser’s team is also developing an API for working with data, called the Neuroscience Data Interface, though it is in the early stages of development.
Challenges ahead
The most often cited concerns around data-sharing and standardization are the time and money it requires. The new regulations specify that applications should include data-associated costs in their grant applications, but the NIH does not have near-term plans to change budget limits for most funding mechanisms. “The most you can ask for without special review is $500,000 in direct costs, and paying a software developer can eat up a big fraction in that,” says Loren Frank, a neuroscientist at the University of California, San Francisco and an SCGB investigator who has helped develop NWB. “I think that will be a challenge going forward.”
Even with available resources, converting data into NWB format is “by no means trivial,” Frank says. Many potential users are unaware of existing infrastructure and conversion packages, and all the options require time, money or both. “It will be particularly tricky for people without a lot of expertise internally,” or resources to hire external help, he says. “There is still a big gap between what needs to be done and what is easy to do.” To help fill this gap, Frank’s team has been building data processing and analysis pipelines that work on top of the NWB format. The tools are available on GitHub, though they are currently in what Frank calls a pre-release stage. “I hope young faculty can take what we’ve done, modify it and save a year or two of writing code,” Frank says.
Standardizing metadata is another major challenge. Platforms may lack a systematic way of logging even simple metadata, such as species name, Van Hooser says. “This metadata problem extends to lots of different types of data, such as electrode types, data formats, behavioral observations, visual stimuli, anatomical structures — the number of ways people refer to anatomical structures is enormous.”
Letisha Wyatt, a neuroscientist and director of Diversity in Research at Oregon Health & Science University, is concerned that the challenges of data-sharing will worsen existing inequities in science funding. “Smaller labs that don’t get giant projects funded or minority scientists who don’t have access to the same level of funding will be in a place where they may not have adequate resources to meet the requirements of this new data-sharing policy in the same way that well-resourced labs might,” she says.
Wyatt is also concerned that little effort is being made to prepare young scientists. “We’ve been having this conversation for a while, and I don’t think we’ve addressed the root of the issue — there is very little formal training for new scientists in this area,” she says. “For graduate students, it’s important they are exposed to effective and rigorous methods for data-sharing throughout their training, so that it’s practiced and familiar when they need it.”
For researchers just starting to think about data-sharing plans, Martone advises focusing initially on how data is stored and shared in their own labs. It’s here that PIs have the most to gain, and these approaches will translate to broader storage and sharing requirements, she says. “These are things we should be doing anyway to make our labs work better.” Simple procedures, such as file naming conventions, can make a big difference. “We rarely think about the next person who will open a file,” she says. “A reasonable person with the relevant skill set should be able to understand it.”
Martone also recommends reaching out to university libraries, which have expertise in managing and cataloging information, and taking online courses in basic data management, such as a University of North Carolina course through Coursera or a FASEB (Federation of American Societies for Experimental Biology) program called DataWorks, designed to support data-sharing and reuse.
Martone, Wyatt and others hope that the community will start to more formally reward effective data-sharing. Researchers are often evaluated on their publication record. But their performance should also be “linked to data-sharing, training and open science practices,” Wyatt says. “We need to reward people who are doing this well.”