
Discoveries of Rotational Dynamics Add to Puzzle of Neural Computation
Your motor cortex is constantly being pulled in different directions. It needs to be flexible, so that you can make subtle changes to your movements, and efficient, so that you can move quickly. Ultimately, it needs to carefully communicate with muscles that are springy and biomechanically complex: one wrong calculation and you might reach past your morning coffee, rather than properly grasping the mug.
For over a century, the dominant idea was that individual motor cortex neurons signaled the strength or direction of a movement, similar to the way visual neurons respond to objects moving left or right. Yet although some motor neurons seem to obey such transparent rules, many others don’t follow this simple mapping. Unlike visual neurons, there doesn’t seem to be a direct correlation between movements and motor neuron activity.
In the past decade, a more dynamic and population-focused model of the motor cortex has evolved. Along with single neurons that represent information by changing their firing rate, neural populations coordinate patterns of output that can flexibly and smoothly command movements. According to this model, motor neuron populations act as dynamical systems: The population’s activity at any moment is governed by its starting point and a rule that dictates how it evolves.
One type of such a dynamical system is a ‘rotational’ one, in which neural populations follow a rotating trajectory through their state space. This rotation can occur in two or more dimensions, but it is most often depicted as a rotation in a two-dimensional plane. Once you have defined the dynamics of the population activity, you can predict the next step. So the most informative aspect of these dynamics is their starting location. By adopting different starting points, the population can generate different types of movements.
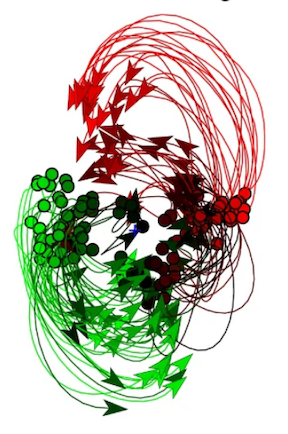
Pandarinath et al. Nature Methods
The concept is similar to that of a record player, where the entire system is always spinning and information arises as the stylus blips over the impressions in the record’s grooves. Perhaps neural population activity is continuously generating rotational dynamics, but as the population wriggles from one groove to the next, it sends different downstream signals — a process that may be much smoother and more precise than individual neurons turning on and off.
In the last year, researchers have uncovered rotational dynamics more broadly in the cortex, in different regions and under different circumstances, prompting speculation that these dynamics could represent a common computational motif deployed by populations of neurons for different purposes.
But at the same time critics continue to question just how meaningful rotational dynamics are. Not every movement is accompanied by rotational dynamics. Furthermore, some researchers claim that finding rotations in the data is a trivial consequence of having consistent sequences of neurons firing.
Amid the criticisms and caveats, one thing is clear: The hunt for rotational dynamics is a powerful framework to compare neural population activity across different brain areas, species and behaviors.
A brief history of rotational dynamics
A new conceptual framework for understanding how the motor cortex controls movement began in 2012, when Mark Churchland and John Cunningham, working with Krishna Shenoy of Stanford, popularized a model of the motor cortex as a rotating system. The idea that the motor cortex may function as a dynamical system was introduced in the literature as early as 1992 but was difficult to test without population recordings. The demonstration of rotational dynamics had to wait two decades, when Churchland and collaborators recorded from enough neurons during a reaching task in monkeys. Churchland and Cunningham (now both at Columbia) and Shenoy are all investigators with the Simons Collaboration on the Global Brain.
Churchland found that individual neural responses during the task were complex and heterogeneous, so he and his colleagues turned to population analyses to make sense of the data. Such high-dimensional population activity — where each neuron is a dimension — can be reduced to fewer variables and represented in a lower-dimensional state space. This approach is broadly known as ‘dimensionality reduction.’ Each moment, the population inhabits a location in that state space and moves to the next spot according to its intrinsic rules.
Using a combination of dimensionality reduction techniques, including their own version of principal components analysis called jPCA, Churchland and colleagues projected their population activity into two dimensions and showed in monkey studies that the rules were rotational. As the animal prepared to move, the jPCA algorithm identified a single axis along which neural population activity aligned. During movement, population activity followed a predictable, rotational trajectory based on its starting point on that axis.
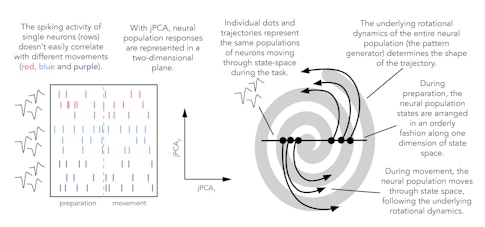
Abigail Russo, a postdoctoral fellow at Princeton University, has a brilliant analogy for these dynamics. Imagine dropping a rubber duck into a whirlpool. Here, the duck represents population activity during a particular movement, and the water represents the underlying rotational dynamics of the motor cortex. The spot where the duck hits the water is the starting point of a neural population’s trajectory. Given this starting point, the duck will follow a predictable, rotational path determined by the water’s whirlpool movement. In the motor cortex, the starting point — which will determine which trajectory activity follows — is tightly related to the type of movement the animal needs to make.
The most impressive aspect of Churchland’s original findings was that the rotational dynamics themselves didn’t depend on the type of reach that the monkey was making. According to Matt Kaufman, a neuroscientist at the University of Chicago who had previously worked with Shenoy and Churchland, the surprise is that all of the conditions obey the same dynamics. “It’s not that you can find a projection where one trace goes in a circle; that’s no surprise to anyone,” he says. “But the fact that they all go in clean circles with any number of conditions, that the rules are basically the same for every movement — that’s surprising.”
The why, when and where of rotational dynamics
Scientists have proposed a few ideas as to why these rotations might be a useful foundation for the motor cortex to build on. They can be simplified computationally and broken down into component parts that can themselves be described with just one or two variables. Fewer variables to encode may mean more efficient computation, and simpler rules may mean easier motor learning. The ability to encode more movement variables with fewer computations enables the motor cortex to flexibly generate many different movements.
Rotational dynamics also offer a smooth trajectory through state space that may translate to smoother muscle activity in the transition between different movements. “Rotations are nice because they don’t have sharp onsets and offsets,” Kaufman says.
The motor cortex may use rotational dynamics to control oscillating pattern generators in the spinal cord, similar to the way gears need to rotate in order to smoothly control other gears. It’s possible that the structural or functional architecture of these networks — quite literally, how they’re wired — leads them to exhibit rotational dynamics.
Lastly, rotational dynamics may help neural networks resist making errors: If nearby neural trajectories always go in similar direction, small mistakes won’t turn into big problems. In 2018, Russo (then a graduate student with Mark Churchland), defined a metric for this ‘tangling,’ and showed that if you add antitangling constraints to neural network models, you can easily end up with loops. “A loop is the simplest untangled thing,” Kaufman notes.
In the past decade, this rotational dynamical model of the motor cortex has been poked and prodded by researchers who would like to know how well it captures neural activity in contexts beyond that of macaques reaching for a target. Since Churchland’s original work with Shenoy, others have shown that recurrent neural networks trained to produce muscle outputs also show rotational dynamics. Follow-up work by Shenoy’s group has since extended their initial findings to reaching in humans.
A recent study from Shenoy and collaborators, published in December 2019 in eLife, has found rotational dynamics underlying speech production, a much more complicated movement than reaching. Sergey Stavisky, a postdoc with Shenoy and Stanford neurosurgeon Jaimie Henderson, and colleagues studied two participants with spinal-cord injuries that left them tetraplegic but still able to speak. The participants had multielectrode arrays placed over the ‘hand knob,’ the part of the motor cortex typically thought of as controlling hand motions. During these recordings, the subjects were asked to move their mouths and tongues, with or without speaking various syllables.
Like previous work with reaching tasks in monkeys, the dynamics of the system were rotational and condition-invariant: Regardless of the content of what the subjects were speaking, their motor networks were following rather simple underlying dynamics. The discovery of rotational dynamics in this system was a surprise, even to Stavisky. “It was one of these ‘Let’s give it a shot’ type[s] of analyses — I gave it a 10 or 20 percent chance of succeeding,” he admits.
Finding rotational dynamics in a human-specific task is a huge boon to the idea that these dynamics are a common computational motif found throughout the evolution of the cortex. “If we think that through evolution and other pressures there is a reuse of successful strategies, maybe that’s what’s happening here,” Stavisky says.
If rotational dynamics are truly a computational motif that has persisted throughout the evolution of various types of cortices and behaviors, we might also expect to find rotations in evolutionarily newer parts of the cortex. And indeed, a preprint released in late 2019 by Mikio Aoi and SCGB investigators Valerio Mante, at the University of Zurich, and Jonathan Pillow, at Princeton University, suggests that rotations can be found in the frontal eye fields in the prefrontal cortex as well.
In their study, Aoi and colleagues extended the potential territory of rotational dynamics to a different context: a perceptual decision-making task in monkeys. Equipped with a new targeted form of dimensionality reduction, Aoi projected population activity onto targeted axes about the stimulus or the animal’s behavior, and stumbled upon rotations. “We weren’t trying to find rotational dynamics,” Aoi says, “We were trying to characterize neural activity by breaking it up into the parts responsible for the encoding of each task variable.” Regardless, their findings suggest that rotational dynamics aren’t restricted to the motor cortex.
It’s not all rotational
Although rotational dynamics may be present during various types of movements and in specific brain areas, they certainly aren’t everywhere. The inconsistency of finding rotations has led some researchers to question the importance of these dynamics, and whether or not they can truly be considered a universal computational motif in the motor cortex.
For instance, a recent preprint from a collaborative effort at the University of Chicago suggests that during more dexterous movements such as grasping, motor cortex activity shows weaker underlying dynamics. Instead, activity in these populations is likely higher-dimensional, or possibly even nonlinear, demonstrating either chaotic, unpredictable behavior, or dynamics more similar to a sensory area. Other networks exhibit attractor dynamics, where the state of the network can realign itself even after being pushed off course, similar to a boomerang.
Different cortical regions may generate and exhibit rotational dynamics, and the dynamics may be more or less powerful, depending on the region. In Russo’s paper, the motor cortex was strongly dominated by internal dynamics, whereas sensory cortices were more strongly driven by external forces. In other words, the motor cortex demonstrates generative dynamics, whereas the visual cortex is very responsive to inputs — a little kid reaching in to grab the rubber duck, if you will.
Furthermore, the fact that neural prosthetics have historically had success despite their rather representational, not dynamical, readout of motor cortex activity raises some questions about the importance of these dynamics. However, a 2017 paper from Shenoy’s lab showed that incorporating dynamics into brain-computer interfaces could modestly improve these device’s lifetimes, potentially improving clinical outcomes for future patients. Including network dynamics in these prosthetics may not be strictly necessary, but it does seem to help.
It’s also possible that even when they are found, rotational dynamics may be a common motif in a neural population, not necessarily a meaningful signal. As a group led by Duke University scientist Miguel Nicolelis has pointed out, a low-dimensional projection of recordings from a population of neurons that are firing in a sequence will indeed look rotational. Similarly, jPCA might return curved trajectories if separate groups of neurons turn on and off in response to different conditions, or if they slowly change the strength of their response to stimulus variables.
For Aoi, these considerations don’t reduce the importance of rotational dynamics when they are found. “I don’t think of this necessarily as a retort to the rotation story,” Aoi says. “It’s just that you have to be more careful about when you observe rotations: Is it because it’s a sequence?” To be sure the rotations aren’t an artifact, researchers can shuffle their data across time or conditions or apply more advanced techniques, such as those developed by Cunningham and Gamaleldin Elsayed, now at Google Brain.
Regardless, the dynamical framework enables researchers to translate findings from one patch of cortex or even from one species to other brain areas and species. “The tools and insight we develop from one dataset, and maybe even from studying the dynamics in artificial networks, can help us learn about how neural networks work generally and can generate all sorts of hypotheses,” Russo says. “This is what is so exciting about this framework.”
For some researchers, the intrigue isn’t necessarily about the rotational aspect of these findings but rather that dynamical patterns exist at all. The discovery of any type of population-level computational motif advances the idea that the motor cortex doesn’t operate via information representation in single neurons. “The picture that’s emerging is that dynamical motifs are consistently and prevalently observed,” says Russo, even if they’re not always like ducks in a whirlpool.
The future of rotational dynamics
In the end, single cortical neurons are likely still carrying important information, but our theoretical frameworks will need to be updated to reflect the dynamical nature of these networks. Within a population of cells that demonstrate rotational dynamics, there are likely single cells that create the patterns alongside cells that simply step in line. Perhaps the activity of single cells will be best understood in relation to their surrounding population. “Is that neuron driving the dynamics? Is it a slave to the dynamics? Or does it just have a preferred, even nonlinear place in the dynamics?” Kaufman asks. “Those are questions that we don’t currently have the tools to distinguish. Not just experimentally, but intellectually.” Understanding the relationship of single cells to the surrounding population can help close the conceptual loop between early work on single cells in the motor cortex and these new population-centered ideas. They might even help us understand why so many motor cortex cells seem to act idiosyncratically. It’s these types of questions that keep Kaufman up at night, and will likely define the next decade of research in this field.
Other researchers are interested in experimentally determining the importance of rotational dynamics. “More work should be done after the fact to understand whether or not these rotations matter,” Aoi says. For instance, do the particular state-space trajectories taken on individual trials correlate with behavior? Aoi found that the radius of the rotation correlated with parameters of the task, “but that doesn’t mean that there’s anything causal about it,” he says. More work is needed to understand whether or not these dynamics are actually necessary for behavior.
Shenoy’s team continues to explore how broadly rotational dynamics are found across the brain, and hopes to record from the speech area directly. Seeing rotational dynamics there would support the idea that rotational dynamics are a common strategy for cortical computation. “Whereas if we don’t [those results],” Stavisky ponders, “it might just be peculiar for forelimb dynamics, and maybe we’re just seeing echoes of that.”
Someday this work might even lead to speech prosthetics that incorporate these dynamics to read out spoken syllables, even from other areas of the cortex. “It’s very hard to develop speech prostheses, because there is no animal model,” Stavisky explains. “But if we have these signals in the hand area, where there are already recording arrays, we can start prototyping decoders to restore speech.”