Deciphering the Secrets of Attention
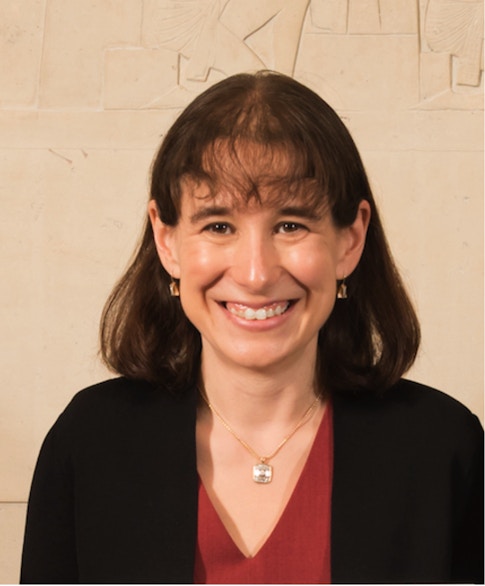
When you walk through the woods, how you perceive your surroundings will vary based on your state of mind — if you’re ardently scanning the ground for mushrooms rather than simply daydreaming your way through a soothing tunnel of trees, you’re much more likely to spot that prized chanterelle.
Marlene Cohen, a neuroscientist at the University of Pittsburgh and an investigator with the Simons Collaboration on the Global Brain, deciphers the neural mechanisms that enable this kind of seamless switch between intent external focus and internal rumination.
Cohen is a pioneer in studying groups of neurons at the single-cell level as animals change their focus, an approach that has provided key insights into our understanding of attention. Based on these studies, she proposes that attention enhances vision by making each neuron act more independently of its neighbors. A group of independently acting neurons can encode more information than a group of highly correlated neurons, enabling the animal to see better. (For more on this, see ‘For Better Vision, Independent Neurons May Be Key.’)
In the last few years, Cohen has expanded her efforts even more, moving from studying groups of neurons in one part of the brain to working with groups of neurons in multiple regions simultaneously. Understanding how groups of neurons communicate — and how those interactions change based on the animal’s needs — will offer new insight into how neural circuits compute.
Cohen, who recently joined SCGB’s executive committee, spoke with the SCGB about her discoveries and her approach to collaboration. An edited version of the conversation follows.
You pioneered efforts to study attention by looking at groups of neurons. What are the biggest insights that arose from measuring interactions among many neurons rather than single neurons or pairs?
My graduate thesis was to record from two cells at the same time by gluing two electrodes together. Luckily the technology has improved. Even going from one neuron to two, it’s easy to see that when you look at changes in internal state, such as attention, a lot of what changed was the interactions between cells. That was not possible to see in single neurons.
When you record from only one neuron at a time, you end up designing the experiment around one neuron. You have to pick a visual stimulus that is ideal for that neuron and to average that neuron’s behavior over many trials. But animals don’t make decisions by looking at the same thing 20 times and taking the average of the best neuron. We make quick decisions based on thousands of neurons in multiple areas. When you record from lots of neurons, you can study something that’s much more like natural behavior — it gives a snapshot of what a subject is seeing or doing at any given moment. The biggest advantage is that it allows you to understand individual decisions or trials or moments in time. It also lets you measure interactions between neurons. In our earliest efforts, we often looked at interactions among neurons in the same area. Now we’re looking at interactions in different areas as well.
When we think about mechanisms, it turns out that single-neuron responses are not effective for constraining mechanistic models. In my collaboration with Brent Doiron [also an SCGB investigator at Pittsburgh], we found that measurements of interactions among neurons in the same area were strong constraints on his mechanistic models. As time moved on, we found that interactions between areas constrained those models even more. I think that more information about how subgroups of neurons interact and how those interactions change with different situations will be the thing that helps us understand the mechanism underlying cognition.
Understanding how different parts of the brain communicate is central to your work and to the SCGB. Why is this such an important issue?
We’ve known for a long time that the organization of the brain is amazing. But hard-wired anatomy is not the whole story. What always attracted me to neuroscience was how flexible the brain is. We can use sensory information in different ways to react to different contexts, to make different decisions in different situations based on some long-held memory. From a functional perspective, information coming from one group of neurons to another has to be flexible. By understanding the general principles of how two groups of neurons communicate and how that changes based on the situation, we’ll learn something important about neural computations.
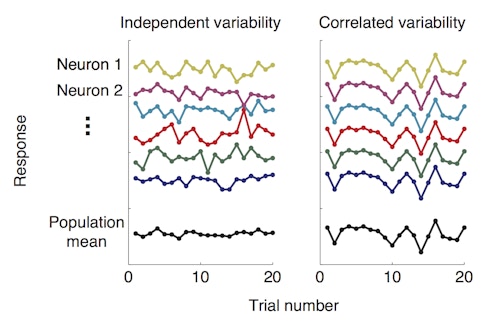
Why is studying attention a good way to start deciphering communication between brain areas?
I think attention is one of a class of cognitive processes that are good for this. We design experiments to have the same visual stimulus and the same basic task structure. But we give the subject two different sets of instructions, which lead to different behaviors. Something must change in the brain to lead to the change in behavior.
Attention is particularly good for studying inter-area communication because in the earliest stages of processing, visual signals are unaffected by attention. But both behavior and motor signals in the brain are affected by attention. So something must be causing that change — that’s what we want to understand.
What are the biggest challenges in understanding how brain regions communicate?
The major challenge is recording from subsets of neurons in the brain during simple behaviors. Our ability to infer transformation from neuronal signals to observed behavior is poor, statistically speaking. Going forward, we will solve that in different ways. One new way is to record from more neurons, but that only gets you so far. An important advance is the ability to train animals to do more complex behaviors. Understanding what combination of neurons allows us to predict a richer set of choices will allow us to nail down how different groups of neurons contribute to behavior and how the brain allows behavior to be so flexible.
You’re involved in a number of collaborations with theorists, both within and outside the SCGB. What makes for a fruitful collaboration?
The traditional model is that you do an experiment and then hand off your data to someone else to interpret or understand it. But I think a lot gets lost in that handoff. I like a more interactive approach. My collaboration with Brent, which has been one of the most long-running collaborations in my own lab, has been useful because we are interested in similar broad scientific questions but use very different approaches to get there. Our approaches have complementary strengths and weaknesses. His models have helped us understand our data in a qualitatively different way. And I think our data helped him constrain his models in a qualitatively different way. It took a lot of time and effort for us to understand the problems in our research and how our efforts might be combined to solve them. It’s been incredibly useful, but you can only have a few of those kinds of collaborations because it’s so time-intensive.
Other kinds of collaborations can be fruitful as well. For example, I went to a theorist’s talk at a conference and realized we had data on our hard drive that could be used to test their hypotheses. That collaboration still needed a lot of interaction to make sure we understood their needs and they understood our data. But the collaboration worked because it began with a well-formed question.
I think collaborations work better when they are longer-term and include true interactions. For example, our SCGB collaboration includes theorists, experimentalists and data analysts, but no one person is doing only one of those things.
Do you have suggestions for promoting collaborations between theorists and experimentalists?
Modern neuroscientific data is often very rich. We design experiments to ask a specific question, but there is always more that can be done with that data. It would be useful to experimentalists to know what other people are thinking about. If I find out a theorist has a specific interest, it might be straightforward to figure out if I have the data to answer those questions. I’d love to have a forum where theorists make the first move, publicizing their directions, questions and needs.
I think this happens already on a small scale. My group has joint lab meetings with other groups as part of SCGB and other communities I’m involved in, and I hear about what theorists have ongoing. I wonder if there is opportunity for publicizing those questions and needs more broadly, in a way that’s easier to comb through on a larger scale.
I and others have been thinking about how to improve diversity in the field and how to connect people who are not already connected. I have a small group of colleagues that I’ve known for a long time. I would love to expand that group and hear what people in both scientifically and physically distant groups are working on.
With the Black Lives Matter movement, pressure to expand diversity in science has taken on new urgency. How can the field address this issue?
Diversity is one of science’s greatest strengths, and racism and limits on immigration are the greatest threats facing American science. We should all be motivated to change things. The data are so firmly on the side of diversity in science being a good thing. In the media, people focus on equality and fairness, which is important. But it’s a scientifically proven fact that to do good science, you need a diversity of ideas. The way you get that is a diversity of people. So this is about fairness and equality, but also about making our science better. There is a lot of important work to do.
One of the groups I have been involved with is BiasWatchNeuro, which has focused mostly on gender equality in science. Scientists respond well to data, so the idea is to compile data on the percentage of women in different subfields and then look at representation of women in high-impact situations, such as giving keynotes at conferences and publishing in high-profile journals. Just publicizing those numbers — say there are 32 percent women in a particular subfield but only 12 percent of speakers at an event in that field are women — has had a measurable effect. The proportion of women at conferences has gone up in ways that have been traceable to BiasWatchNeuro. We are hoping to expand these efforts to other groups. But increasing racial diversity will require a combination of many factors. The numbers of investigators are so low, just publicizing them won’t be enough. I hope that all scientists will come together to improve our community and our science.
Another effort I’m especially excited about is a new master’s-to-Ph.D. bridge program in bioengineering and neuroscience for students from underrepresented backgrounds that my colleague Gelsy Torres-Oviedo at the University of Pittsburgh designed. Her approach is data-driven: She has researched how to best identify promising students, get them interested in research, make sure they know how welcome they are here, and give them the technical and professional skills to have a career in research. I hope this program will produce a cohort of promising scientists who bring much-needed perspectives to our field.
What advice do you have for young neuroscientists?
I think the right way to do science is to do it your way. When I was a trainee, I looked for examples of what successful scientists looked like — what they spent time on, what they prioritized. It’s important to learn from other people, and I still try to do that. But I would do a bad job trying to model my career after one model of success. I needed to find questions, techniques and a style that worked for me. I do my best work when I am focusing on things that are a good match for me.