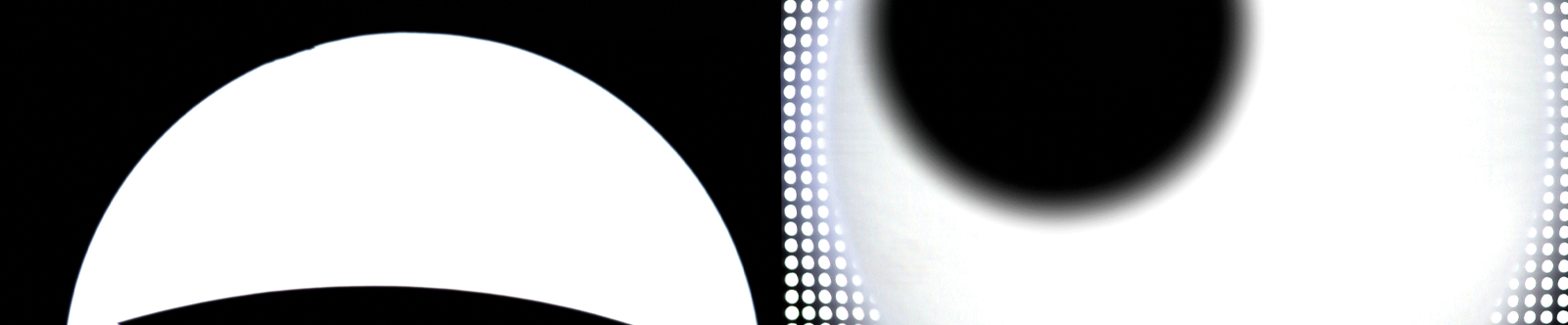
Deconstructing Machine Learning’s Black Box
Artificial intelligence has taken the tech world by storm. AI systems can drive cars, recognize faces and even dethrone world champions at games such as Go. At the Flatiron Institute, scientists are leveraging the same techniques to simulate the universe, track neuron activity in the brain and predict the behavior of many entangled electrons.
A fundamental problem lingers, though. Machine-learning algorithms such as neural networks “are black boxes,” says Stéphane Mallat, distinguished research scientist at the Flatiron Institute’s Center for Computational Mathematics (CCM). “They work well, but we don’t know what’s being learned. It doesn’t help us understand the phenomena.”
Machine-learning models ‘learn’ by ingesting large amounts of example training data. After training, the models can produce results not possible through conventional methods. The inputs and outputs of such a model are clear but what’s happening in between remains mysterious.
The tech companies driving machine-learning development, including Google and Facebook, seem more focused on results than deeper understanding. “Right now, machine learning is very much an empirical field,” Mallat says. “There are many algorithms which are working well, but we don’t understand what type of structures they learn and the mathematics behind them. This means that we cannot interpret results or guarantee their robustness.”
At the CCM, researchers are working to reverse engineer solutions from machine-learning applications to figure out what led to the result. Using that information, the researchers hope to learn more about real-world systems and improve conventional methods. The CCM hosts regular meetups in which researchers from all four centers discuss the latest developments in the field and talk about their machine-learning projects.
“There’s a need for new mathematics in this area,” Mallat says.