A Step Toward Mind Reading: Decoding Perceptual Decisions as They Unfold
Imagine a chess player at a tournament deliberating on her next move. She is likely weighing the optimal strategy given her prior experience, her opponent’s style and many other variables. During that thought process, she might cycle through a series of possible decisions, with each choice quickly updated as she considers new information — a memory of a previous move by this opponent, for example.
Scientists would like to understand how the brain makes these kinds of calculations. But it’s a challenging problem. The decision states are all reflected in the player’s neural activity. But they’re difficult to study given that these patterns are not tied to any outward behavior — the outcome of her deliberation becomes clear only when she finally moves the piece.
Neuroscientists typically study decision making in the lab using highly constrained tasks — an animal might have to match two similar images or decide on the dominant color or motion of a set of moving dots. Even these simple tasks are unexpectedly complex, however. The brain can respond differently to the same image because of fluctuations in the animal’s state of mind, such as whether it’s paying attention to the image. That variability in turn can influence behavior. If the stimuli are ambiguous enough — if roughly half the dots are red and half green when the goal is to report the dominant color — subjects will make different choices on different trials even if the stimulus is exactly the same.
For several decades, systems neuroscience, and decision-making research in particular, dealt with this variability by repeating the same or nearly identical stimuli over and over. Averaging neural responses from one or a few neurons across many such trials isolates the consistent responses. This approach has had great success and has vastly improved our understanding of the neural underpinnings of many phenomena, including vision, attention, decision making and spatial navigation. But it discards variability across trials and even within trials. That information is crucial for understanding non-repeatable processes, such as the internal deliberation that both the lab subject and the chess player go through before reaching a decision.
In fact, we know from our own subjective experience that most of our cognitive life is not repeatable or necessarily dependent on external events. To understand these cognitive processes, we must leave the trial-averaging approach behind and build tools to decode and probe internal cognitive states.
In the last five to 10 years, multiple research groups have taken on this challenge.1-4 The most common approach has been to simultaneously record from large populations of neurons and use that information to predict what an animal will decide well before it reveals its choice. The animal’s decision state can be defined as the likelihood at that point in time of its choosing each of the options available.
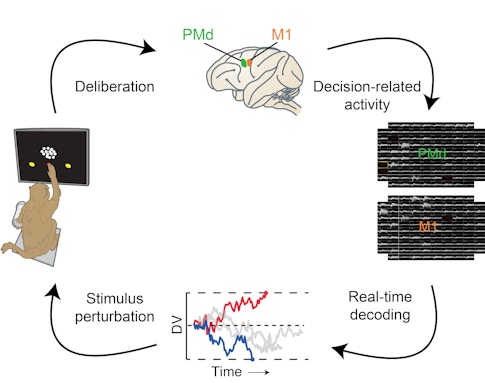
Scientists typically estimate decision states after the fact by analyzing data collected during the experiment and grouping trials together that have similar neural activity patterns. We took the same basic approach for collecting data, recording from up to 200 neurons in the dorsal premotor cortex and the primary motor cortex in monkeys as they watched a video of dots moving left and right. However, we went one step further: Instead of estimating decision states after the experiment, we calculated them in real time as the animal performed the task. This way we could almost instantly (within 25 milliseconds) make an educated guess about whether the monkey was going to choose left or right and then prompt the animal to report its decision, verifying if our estimate was correct. Our approach was inspired by the decoding framework used for brain-machine interfaces.5 Designing the setup and implementing it was a joint effort of the Newsome and Shenoy labs at Stanford University.
We had learned in a previous project that, on average, signals in the dorsal premotor cortex and primary motor cortex reflect the animal’s evolving deliberation. That is, these signals have the properties of a time-varying decision variable: They do not predict the animal’s choice before the stimulus is presented, they rise faster for easier trials, and they are slightly stronger before the animal makes a correct choice than when it’s about to make an error. Together, these properties are evidence that the signals convey both the animal’s decision and the confidence associated with it.
Within an individual trial, however, we see large moment-to-moment fluctuations of the decision variable. The real-time setup allowed us to examine whether these fluctuations faithfully reflect the ongoing decision process or if they simply result from our inability to estimate reliably. To test this idea, we estimated the decision variable every 10 milliseconds using 50 milliseconds of spiking data from the two brain regions of interest. We verified that the decision variable could accurately predict the animal’s choice well before it was unveiled — for stimuli longer than 600 milliseconds, the decision variable’s prediction was correct more than 90 percent of the time.
We then performed two closed-loop experiments in which we stopped showing the monkey the moving dots based on the magnitude or history of the decision variable and asked the animal to report its decision.
In the first experiment, we stopped the stimulus at five randomly chosen magnitudes. The higher the magnitude, the more likely the animal was to make a given choice (right or left). Even though our predictions relied on only 50 milliseconds of neural data from 100 to 200 neurons, we found that the average difference between the empirical and the predicted likelihood of a given choice was less than 2 percent. This was true across a wide range of decision-variable magnitudes, across different stimulus durations and difficulty levels, and even across multiple sessions. The results show that we can decode moment-to-moment fluctuations in the animal’s decision in real time from the dorsal premotor cortex and primary motor cortex.
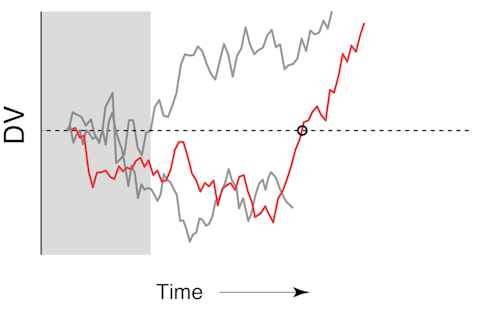
In the second experiment, we stopped the stimulus when the decision variable fluctuated from a likely left choice to likely right. We theorized that these changes — which are fairly rare, occurring in only 5 to 10 percent of trials — reflect the animal changing its mind. We found that the decision variable following this putative change of mind predicted choice nearly as accurately as it did in trials with no change of mind, demonstrating that even for these rare and unusual trials our decoder was very accurate in predicting the animal’s ultimate choice. In addition, features of these putative changes of mind followed the pattern of those detected in humans performing an identical task6: They were more frequent in harder trials, more likely to correct a mistake than to lead to an erroneous choice, and more likely to happen earlier in the trial.
These results suggest that large and robust fluctuations in the decision variable do reflect the cognitive process of a change of mind. The real-time setup was particularly crucial for this experiment by allowing us to capture every single trial where the animal seemed to change its mind. Because these trials are rare, an analysis with similar experimental controls using offline methods would require a prohibitively large number of trials.
We are now working on a third closed-loop experiment in which, instead of stopping the stimulus based on neural state, we slightly perturb the stimulus to make the direction of motion clearer or noisier. Understanding how the brain responds to these changes as a function of time and with respect to current and past decisions will allow us to probe the dynamics of decision-related activity in the dorsal premotor cortex and primary motor cortex in a systematic manner and test predictions from different theoretical models.
Decoding the decision variable in real time grants us access to brain processes, such as decision making, that are typically hidden. We believe this approach can be extended to other types of decision-making processes and other covert phenomena such as attention.
Diogo Peixoto is a postdoctoral researcher in the Newsome and Shenoy labs, and Jessica Verhein is a doctoral student in the Shenoy lab.
References
1. Bollimunta A, Totten D, Ditterich J. Neural dynamics of choice: single-trial analysis of decision-related activity in parietal cortex. J Neurosci. 2012;32(37):12684-12701. doi:10.1523/JNEUROSCI.5752-11.2012.
2. Kaufman MT, Churchland AK. Many paths from state to state. Nat Neurosci. 2016;19(12):1541-1542. doi:10.1038/nn.4440.
3. Kiani R, Cueva CJ, Reppas JB, Newsome WT. Dynamics of neural population responses in prefrontal cortex indicate changes of mind on single trials. Curr Biol. 2014;24(13):1542-1547. doi:10.1016/j.cub.2014.05.049.
4. Rich EL, Wallis JD. Decoding subjective decisions from orbitofrontal cortex. Nat Neurosci. 2016;19(7):973-980. doi:10.1038/nn.4320.
5. Bensmaia SJ, Miller LE. Restoring sensorimotor function through intracortical interfaces: progress and looming challenges. Nat Rev Neurosci. 2014;15(5):313-325. doi:10.1038/nrn3724.
6. Resulaj A, Kiani R, Wolpert DM, Shadlen MN. Changes of mind in decision-making. Nature. 2009;461(7261):263-266. doi:10.1038/nature08275.