Why Sex?
At the heart of the theory of evolution lies a conundrum.
Most people think of natural selection in terms of “survival of the fittest.” And when it comes to asexual organisms, that catchphrase is pretty much on the mark. The fittest individual in a population will (by definition) have the most offspring, and over generations, the number of these offspring—all identical to their common ancestor—will grow exponentially and gradually take over the population, crowding out less fit individuals.
When it comes to sexual reproduction, however, the fittest genotypes do not survive down the generations. If nature comes up with an exceptionally fit individual, there is no way to preserve that individual’s makeup intact in the next generation. Each offspring gets only half of the fit parent’s genes, and over generations, that parent’s contribution becomes more and more diluted. Instead of hanging on to the winning hands, sexual reproduction shuffles the deck with each generation, seemingly driving the population towards a steady mediocrity. On the face of it, sexual reproduction seems like a pretty stupid idea.
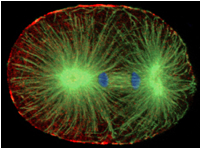
And yet, sex is everywhere. In nature, it is the asexual species that are the exception: almost all species reproduce sexually at least part of the time. The few known species that can reproduce only asexually are believed by scientists to have evolved “by accident” from sexual species, and are seen as evolutionary dead ends.
If sex is so ubiquitous then it must be good for something. But what? Over the years, evolutionary biologists have proposed various answers to this question, often called “the queen of problems in evolutionary biology.” Some argued that sex allows a population to weed out harmful mutations more quickly, or helps a population to evolve faster than its parasites. Each explanation, however, required a different set of restrictive assumptions, and none has achieved wide acceptance.
Now, a team of mathematicians, computer scientists and biologists has proposed a fundamentally new solution to this seeming paradox. Unlike previous attempts at explanation, which offered reasons why sex might be beneficial despite its dilution of the fittest genomes, the team argues that sex is beneficial precisely because of this dilution process.
Sex, the researchers argue, acts like the “good banker” who (hopefully) rebalances your investment portfolio each year. Like sexual reproduction, rebalancing is counterintuitive: you’re supposed to sell winning investments that are taking over your portfolio in order to buy more losers. Yet in the stock market, rebalancing makes good sense. That high-performing stock might not be so hot next year, while the loser might just be poised for a recovery. On average, rebalancing reduces portfolio volatility without damaging returns.
In the sex market, the constant rebalancing favors not the combinations of genes likely to produce the most offspring in the next generation, but the genes that are good mixers, performing well in combination with many different genes. These versatile genes stand the population in good stead in nature’s ever-changing landscape. Sex, the research team says, promotes not the survival of the fittest but something more subtle: the flexibility and robustness that enables a population to roll with the evolutionary punches.
“Everybody had assumed that the whole point of sex is to help increase fitness, but it’s not,” says Christos Papadimitriou, a member of the research team and a computer scientist at the University of California, Berkeley.
In a pair of papers in Proceedings of the National Academy of Sciences in 2008 and January 26 of this year, the researchers show, through simulations, that sexual reproduction does a worse job than asexual reproduction of increasing average population fitness, but a better job of increasing the population’s “mixability”—the ability of its genes to perform well in many different genetic contexts. The researchers have also proved a theorem to this effect, in unpublished work. The new findings have some surprising consequences for the very nature of genes.
The work is an important step forward, says Günter Wagner, an evolutionary biologist at Yale University. “It answers questions that have been open since the 1930s,” the dawn of modern evolutionary theory, he says.
Computer Science Imitates Nature
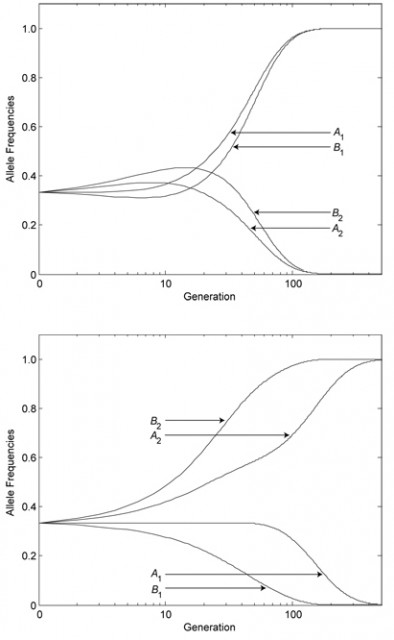
The idea behind the new analysis arose out of a discussion between Papadimitriou and Adi Livnat, a colleague at Berkeley, about a baffling problem in computer science.
Inspired by evolution, computer scientists have created two different types of algorithms that take an approximate solution to a problem and gradually hone it into a better solution. One algorithm, called simulated annealing, mimics asexual reproduction: start with a population of possible solutions, allow them to mutate, let them “reproduce” according to the rule that the best new solutions produce the most offspring, and then repeat the process again and again. The other algorithm, called a genetic algorithm, mimics sexual reproduction: potential solutions are allowed to recombine with each other, and the best solutions get to reproduce the most.
Simulated annealing tends to work quite well, but genetic algorithms do not.
“When computer scientists heard about genetic algorithms their eyes would light up, but after a few months of trying to solve problems in the lab, they were always disappointed,” Papadimitriou says. “It was a paradox – how come nature’s favorite trick falls on its face when done digitally?”
Mulling over this paradox, Livnat and Papadimitriou realized that sex does a second-rate job of optimizing fitness when it comes to solving a fixed, static problem like those encountered in computer science. Instead, where sexual reproduction shines is in circumstances in which the very problem being solved keeps shifting underfoot. In nature, the notion of fitness keeps changing as a species’ environment changes.
To understand how sex solves an ever-shifting problem, the researchers had to bring new mathematical techniques into play. Most studies of evolutionary dynamics focus on how a population’s fitness converges to an equilibrium as the number of generations approaches infinity. But in studying the problem of sex, the behavior approaching infinity is irrelevant: long before the population’s fitness nears equilibrium, the landscape will have shifted and the definition of what makes an organism fit will have changed. To deal with this, the researchers developed tools—both simulations and theoretical approaches—that captured what happens to a species during the little-studied “evolutionary transient,” the stage of evolution in between the first few generations and the equilibrium at infinity. The analysis showed that sex favors not the fittest individuals, but the most flexible genes.
Sex Shapes the Genome

The new work offers an explanation for why asexual species are isolated twigs on the tree of life. Since sex favors genes that can enter into successful partnerships with a wide range of other genes, the resulting species have the flexibility to tolerate the mutations and genetic shuffling that drive evolution. Thus, sexual species can evolve into new species more quickly and robustly than asexual species can. And so the laws of exponential growth dictate that in the long run, sexual species must take over the tree of life.
“Sexual reproduction makes the evolutionary landscape change quickly, and the asexual species that had meticulously optimized the landscape are left looking silly,” Papadimitriou says.
An early form of sex—in the form of shuffling of bits of genetic material—may even have been a driving force behind the formation of genes as we know them today, the researchers suggest. Because of this shuffling, sex rewards genomes that organize themselves into discrete chunks with different functions. Such genomes have a much better chance of producing viable offspring under sexual shuffling than genomes in which every part depends on every other part to function.
In other words, sex favors genomes that are “modular,” like the inside of a car, in which the different components all interact, but it’s possible to replace an individual component without destroying the car. Genetic modularity—in the form of a hierarchy of genes and interacting sets of genes—is key to the formation of complex organisms.
By contrast, in asexual reproduction, the entire genome is preserved intact from one generation to the next, so there is no pressure for the genome to organize itself into modular chunks such as genes.
The new work calls into question the conventional wisdom that asexual reproduction predated sexual reproduction. “If sex is tied to the very nature of genes, then genetic shuffling may have existed in some primordial form very far back in time,” Livnat says.
One question left unanswered by the new research is why sexual reproduction survives in the short-term, even though it is an inefficient and costly way to produce offspring, compared to asexual reproduction, and seems to do a worse job of increasing short-term fitness. The new work offers an explanation for why, if sex survives in the short-term, it should dominate in the long-term, and how it should shape the genome along the way. Explaining the mechanisms that keep sex alive in the short-term despite its costs is an important unsolved problem, Livnat says.
The researchers’ analysis is a novel take on the problem of sex’s role in evolution, says Stephen Stearns, an evolutionary biologist at Yale University. The crucial next step, he says, is for evolutionary biologists to test how well the theory explains empirical findings in genetics research.
It may take experimentalists some time to wrap their heads around the new findings, Stearns says. “These contributions are creative enough to be puzzling,” he says. Concepts such as modularity have a long history in computer science, since modularity is an important aspect of the design of computer programs. But evolutionary biologists are just starting to appreciate its explanatory power, as they gradually unfold the complex structure of genomes.
The ideas offered by the new analysis are probably only part of a more complex picture, cautions Sean Rice, an evolutionary biologist at Texas Tech University in Lubbock. For example, while increasing mixability may benefit a species’ evolvability, another probable way that sex benefits evolvability is simply by bringing new genetic combinations into a population more quickly than mutation alone does. Disentangling the effects of those two contributions to evolvability could be tricky, Rice says.
However, it’s definitely worth exploring the implications of the model further, Rice says, especially if the researchers could add some more biological complexity, such as allowing organisms to reproduce sometimes sexually and sometimes asexually, as happens often in nature. “Adding a bit more complexity could perhaps give something of great use,” Rice says. “They could test the model’s predictions instantly on an immense amount of data from real organisms.”
Understanding the mathematics behind the new approach is well worth the effort for evolutionary biologists, Stearns says. “In a field as mature as evolutionary genetics, it’s not so frequent that someone takes an old problem, looks at it from another angle, and finds new connections,” he says. “I think they’ve done it.”
Ultimately, the research team argues, it is impossible to make sense of how evolution has shaped the genetic architecture without factoring in the role of sex.
“I don’t see how you can tell the story of life without this,” Papadimitriou says. “Sex truly was the original sin.”
Back to main article >
Modeling Modularity
Researchers studying the role of sex in evolution generally use standard models of population genetics that look at how a population’s average fitness changes over the generations. The version of the model that Livnat et al. uses starts with some random initial population of individuals of differing genotypes; each possible genotype is assigned a fitness value, that is, an ability to produce viable offspring. To produce the next generation of the model, every individual is allowed to produce as many offspring as its fitness value dictates. Thus, the model allows researchers to track both a population’s average fitness and which genotypes predominate over time.
In the 1930s, the founders of population genetics used this type of mathematical model to show that even though sexual reproduction breaks down the best gene combinations, a sexual population’s average population fitness still increases over time. “The result was celebrated as the crown jewel of modern evolutionary theory,” Livnat says. “People took it as a proof that Darwin was right—that fitness increases.”
What the early work left unexplained, however, was the fact that sexual reproduction does a worse job of increasing average fitness than asexual reproduction does, given the same initial data. Given that, why does sexual reproduction dominate the tree of life?
To tease out the role of sex in evolution, Papadimitriou, Livnat and their collaborators realized that they must measure not just a population’s average fitness but also its average “mixability”: how well each gene variant is able to combine effectively with a wide range of other gene variants.
Imagine a species with just two genes, A and B, and suppose that gene A comes in n different variants—or alleles—A1,A2, …, An. B comes in m alleles: B1, B2, …, Bm. An allele’s mixability boils down to the degree to which the allele’s contribution to fitness is independent of which other allele it is paired with. To calculate this mixability, the researchers wrote the fitness F of a given phenotype AiBj as the sum of three separate terms, as follows:
F(AiBj) = ai + bj + εij
where ai is a term that depends only on allele Ai, bj is a term that depends only on allele Bj, and εij is a correction term that takes into account the way that the interaction between Ai and Bj impacts fitness. The ε‘s are chosen to be as small as possible (in the sense of least squares), to capture as well as possible the extent to which the different allele’s contributions can be separated out from each other. Once that choice of ε‘s is made, the term ai can be thought of as representing allele Ai‘s separate contribution to fitness, and bj as representing Bj‘s separate contribution to fitness.
If, for a particular i and j, εij is large, then it’s impossible to disentangle the effects of Ai and Bj from each other; the genotype AiBj may be very fit, but its alleles are not good mixers. By contrast, an allele Ai that has low ε‘s with all the different Bj‘s is a good mixer, and has its own independent effect on fitness, regardless of what it is paired with.
The researchers define a genotype’s mixability M as
M(AiBj) = ai + bj.
As an example, consider a population with three alleles of gene A—A1, A2, and A3—and three alleles of gene B—B1, B2, and B3—and the following fitness matrix:
A1 | A2 | A3 | |
B1 | 7 | 1 | 4 |
B2 | 1 | 7 | 4 |
B3 | 4 | 4 | 6 |
A quick glance shows that the fittest genomes are A1B1 and A2B2, but the alleles A1, B1, A2, and B2 don’t perform so well when broken out of their best combinations. By contrast, A3 and B3 are good mixers, offering a reliable, but not spectacular, level of fitness regardless of what alleles they are paired with. The mixability measures of the different genotypes can be approximated as follows:
ai | bj | εij | Fij | Mij | |
A1B1 | 0 | 0 | 7 | 7 | 0 |
A1B2 | 0 | 0 | 1 | 1 | 0 |
A1B3 | 0 | 3 | 1 | 4 | 3 |
A2B1 | 0 | 0 | 1 | 1 | 0 |
A2B2 | 0 | 0 | 7 | 7 | 0 |
A2B3 | 0 | 3 | 1 | 4 | 3 |
A3B1 | 3 | 0 | 1 | 4 | 3 |
A3B2 | 3 | 0 | 1 | 4 | 3 |
A3B3 | 3 | 3 | 0 | 6 | 6 |
The researchers found in their simulations that, over the generations, a sexual population’s average mixability grows steadily. In the above example, in an asexual species, A1B1 and A2B2 would eventually dominate the population. By contrast, in a sexual species, alleles such as A3 and B3 prevail over time, to the slight detriment of fitness but the benefit of mixability.
In species with more than two genes, it is fruitful to consider not just the mixability measure but also higher-order measures that take into account the contributions to fitness from pairs, triples, or larger collections of genes. For example, in a species with three genes, A, B, and C, that each come in several varieties, we could write
M2(AiBjCk) = ai + bj + ck + rij + sjk + tik,
where ai, bj, and ck represent the separate contributions to fitness of each gene, and rij, sjk, and tik represent the contributions to fitness of the pairs AiBj, BjCk, and AiCk, respectively. In general, given a species with n genes, there is a sequence of measures, M1, …, Mn, where M1 is the mixability measure and Mn is the actual fitness. These measures give a glimpse of higher-order modularity in the genome: Mj, for example, measures the extent to which “modules” with jor fewer genes make contributions to fitness that are independent of what’s going on in the rest of the genome.
In their simulations, the researchers found that the lower-order measures increase robustly in sexual species, showing that sex favors not only individual “good-mixer” genes but also good-mixer modules of several genes working together. For higher-order measures, close to the full fitness measure, the advantage of sex over asex becomes blurred—not because asex favors any modules smaller than the whole genome, Livnat says, but because of the coarseness of the measures.
Figuring out at which levels the genome possesses the highest degree of modularity is a question of great biological significance, Livnat says. “Our theory gives us a heuristic argument for the idea that it’s not necessarily the single genes that have the most clearly demarcated separate effects on fitness, but it would take advanced mathematical techniques to make these ideas rigorous,” he says.