Lea Duncker, Ph.D.
Postdoctoral Fellow, Stanford University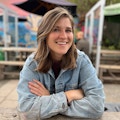
Lea Duncker is a postdoctoral researcher at Stanford University, where she works with Krishna Shenoy and Scott Linderman. She has a bachelor of science in natural sciences and a master of science in computational statistics and machine learning, both from University College London. Duncker obtained her Ph.D. at the Gatsby Computational Neuroscience Unit under the supervision of Maneesh Sahani. Her research focuses on characterizing and interpreting population-level structure in neural data, with a focus on dynamical systems and motor control. In her future research, Duncker is planning to establish a joint theoretical research group in collaboration with Laura Driscoll. Their work will investigate how neural populations flexibly perform computation under varying task demands, and how the structure facilitating this changes throughout learning. Duncker and Driscoll view a joint-group structure as an opportunity to engage in collaborative science and to create a stimulating and inclusive environment for doing academic research.
Project: The dynamics of flexible computation and continual learning
Animals adapt to new environments throughout their lifetimes, flexibly acquiring and deploying new skills and knowledge. Yet, little is known about how the recurrent neural circuits that create behavior are able to learn, store, choose between and express multiple patterns of action without interference. Duncker’s future group will investigate: (1) the general organizational principles of network dynamics to support flexible computation, (2) how new structure is incorporated into this organization to support continual learning, and (3) how computational hypotheses can be tested experimentally using targeted circuit perturbations. Addressing these questions will be made possible through methodological advances, combining mechanistic insights from dynamical systems theory and artificial network modeling with neural data via interpretable statistical models. This work will aim to establish direct links between neural data, computational model hypotheses, and theoretical properties of neural circuits. Overall, this work will contribute to a more fundamental understanding of the neural mechanisms underlying flexible behavior and continual learning.