Building Computer Models That See Everything
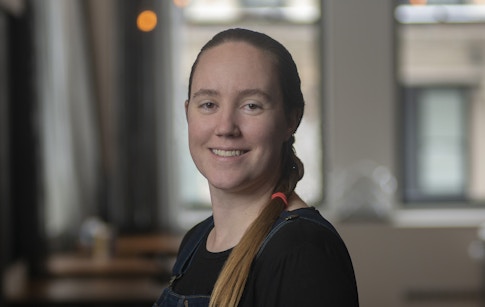
The more scientists learn about the mechanics of sight, the more complicated it gets. Acting as a sort of high-powered computer, the visual system can take in what is directly in front of us, what’s at the edge of our peripheral vision, and what’s at our feet when we are walking — all simultaneously and seemingly in real time. But neuroscientists do not yet have a full understanding of what is happening inside the brain as we see the world.
Simons Junior Fellow Kathryn (Kate) Bonnen, a postdoctoral scholar at New York University who also has an appointment at the Flatiron Institute’s newly opened Center for Computational Neuroscience (CCN), is working to change that. Many of the classical models for understanding vision, while helpful, are limited to two dimensions and can’t fully account for our visual experiences. Bonnen, whose fellowship will conclude this year, is working to build more robust models for a 3D world.
Bonnen’s adviser at NYU is Eero Simoncelli, who is also the CCN’s founding director. Bonnen holds a Ph.D. in neuroscience from the University of Texas at Austin.
We recently spoke about how her work can lead us to a deeper understanding of the visual system. Our conversation has been edited for clarity.
You study vision and are also part of the Flatiron Institute’s new Center for Computational Neuroscience. What is computational about your work?
I work to understand the neuroscience of visual perception: the mechanics of what goes on in the brain as we perceive the world. The field of visual perception actually has deep computational roots, having relied on foundational research on radars and signal detection performed by engineers during World War II. In the years since, engineers, mathematicians and physicists have all shaped the field.
These days our efforts focus on building computational models that can describe the relationships between what our eyes see and the subsequent neural signals that give us our complete visual experience. These models do a good job of describing relationships when the visual input is very simple, when it can be shown in two dimensions on a computer screen. For example, we have a very good understanding of how the eyes and brain work when, say, a series of dots move up and down or left and right. But most of what we perceive in the real world is more complicated than that. I want to understand what happens during these more complex visual experiences.
One nice thing about studying vision — compared to, perhaps, the study of memory or emotions — is that we can measure visual input deeply and in rich detail, and then see how the brain processes that input. This makes vision a good gateway to understanding fundamental brain function, which is of course the goal of every neuroscientist regardless of their specialty.
I like that: vision research as the gateway to understanding how the brain functions. With that in mind, tell me more about your particular focus.
I focus on how we see in the real world during natural behavior. This involves thinking about depth and how our visual experience changes over time, which is trickier than you might expect. With a 2D image, like a photograph, there’s a direct connection between the visual image and the back of the retina, but with 3D objects the brain has to reconstruct that 3D image in the brain. We’re continuing to learn how this happens.
In graduate school I helped discover that the part of the visual cortex that helps us understand simple 2D motion is still involved when we perceive more complex 3D motion, though in a strikingly different way. For decades, neuroscientists had expected that 2D and 3D visual processes would operate similarly, just at different levels of complexity and perhaps in different areas of the brain. What we’ve since discovered is that while this brain region does carry information about both two and three dimensions, the processes are quite different when there’s depth involved. This was unexpected.
So those early expectations were not wrong, by any means. They produced a logical hypothesis about 3D visual processing that could have been proved by future experiments. How was that hypothesis tested in more recent years?
Scientists had long assumed that 3D visual processing would ‘look like’ 2D visual processing in the brain, but we now know that is not always the case.
One of the first clues that we needed to think about 3D motion differently actually came from perceptual experiments. No fancy equipment or neural recordings, just a computer monitor and a person to answer questions about what they could see. In the experiment, people were asked to estimate the direction of motion that they saw. They used a pointer to indicate which direction the dots on the screen were moving. And when we made the motion hard to see, people made funny mistakes, such as seeing dots coming at them when they were actually going away (or vice versa). At first the mistakes were hard to understand, but these mistakes turned out to be the clue that solved the mystery.
Even though the directions described by participants were quite different from reality, the mix-ups showed us that those directions are close to each other in ‘perceptual space.’ The question then became: How does the brain implement motion perception in a way that results in those perceptual mistakes? When I started looking at the relevant neural data, I noticed that the neural responses also looked different than we expected. But the patterns in the neural data were still systematic, so I built a computational model that could mimic the neural data. I didn’t use any of the perceptual data to design this model, but when we used the model to make predictions for motion perception, our model made the same perceptual mistakes even though it had not been primed to do so. So we were able to use this model to connect the neural data to human motion perception.
What are some practical implications of your work?
First of all, the better we understand what happens during complex visual processing, the more we can build tools to help people see better — or even build tools like robots that can perform complex visual tasks. Right now, I think it’s fair to say that the biomechanics of such robots are more developed than their visual faculties, but my vision science work could contribute some interesting ideas for how to coordinate biomechanics with computer vision.
I am also excited to see how continued advances can help coordinate visual perception with movement — such as how we use vision when we’re hiking, when we depend on our visual perception to safely navigate often uneven terrain.
For example, after earning my doctorate and just before coming to NYU, I completed a brief postdoc at the University of California, Berkeley’s School of Optometry. In collaboration with a biomechanics expert, I studied how different kinds of visual impairments affect the way people walk.
Part of this work involved outfitting people with body motion sensors and cameras as they walked through the hills near the UC Berkeley campus. These cameras can capture what people see as they walk, and also exactly when their eyes fall on the ground. When combined with our knowledge of neural data, this real-world dataset is a trove for better understanding what happens as we move through the world in real time. It’s been one of the key datasets of my NYU postdoc and Simons fellowship.
That sounds fascinating! What’s next for you, after the fellowship ends?
Both my postdoc and the fellowship end in December, and in January I’ll begin a faculty position in the Indiana University School of Optometry. One of my first tasks will be to teach visual neuroscience to first-year optometry students. I’m excited!
Finally, what are your thoughts about the Simons fellowship?
The fellowship really does prepare you to be part of a much larger scientific community, in a way that most of my training didn’t.
As a graduate student and postdoctoral researcher, you spend your time with other people who are very focused on your same area of research: other neuroscientists. That builds camaraderie and deep expertise but is a bit insular too. Simons Fellows come from many fields — from neuroscience to mathematics to immunology — and at our regular dinners you might sit down next to one of the world’s most respected astrophysicists. When they ask you about your research, you can’t use the field-specific jargon or shortcuts anymore. Instead, you have to explain things with scientific precision but in plain language. That was wonderful training and experience, which I’ll always take with me.