Q&A: The Battle Between Learning and Memory
If you’re standing at an intersection trying to hail a cab, you’ll likely pay attention to the colors of the oncoming cars. But if you’re trying to cross the street safely, you’ll probably ignore the colors and instead focus on the speed and position of the closest car. These largely unconscious choices reflect the sum of many past experiences, which the brain has somehow organized to help us pick the most important factors — color versus speed. This structure built from past experiences allows us to make the best choices in everyday life without a second thought, whether we’re crossing the street or hailing a cab.
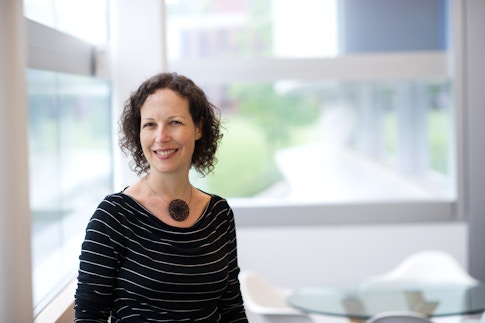
Yael Niv, a neuroscientist at Princeton University and co-director of the new Rutgers-Princeton Center for Computational Cognitive Neuro-Psychiatry, explores how the brain creates and organizes these representations. Niv argues that a key feature of learning and memory is figuring out the latent structure of the environment — for example, that the speed of an oncoming car rather than its color is important in determining if it will hit you and thus whether it’s safe to cross the street.
According to Niv, an essential part of learning latent structure is appropriately clustering experiences, grouping similar encounters together or forming new clusters for novel situations. Indeed, Niv describes a sort of battle between learning and memory, where the brain has to decide whether to put new information into an existing cluster — learning by generalizing — or to create a new cluster — memorizing a new set of rules. Her work could have implications not just for understanding how we learn, but for understanding and treating disease, such as post-traumatic stress disorder and schizophrenia.
Niv talked with the SCGB about her theory and some key experiments from her lab. An edited version of the conversation follows.
Your research focuses on how the brain learns to represent the world. What specific questions are you working on?
Day-to-day tasks often come without instructions, so how do we learn the correct representation for each task from trial and error? The ‘correct’ representation is one that reflects the true causal structure of the task. For example, the color of a car doesn’t determine if I’ll get run over, so it has no causal effect on the outcome of the task of crossing the street. What representation of the environment would make it easiest to decide how to execute this task? Also, what representation would make it easiest to generalize learning? If someone honks at me when I try to cross the street, what should I learn from that?
The thing I’ve learned over and over again is that our brain is like a scientist. As scientists, we try to understand what causes what in our domain of research. Our brain naturally does that all the time, trying to figure out what causes what in the world using evidence and ‘experiments’ that we perform on our environment. How does the brain do this, especially when it comes to the difficult task of learning a representation? And how does this process relate to psychopathology? For example, is over- or under-generalization in learning related to PTSD or schizophrenia? To answer these questions, we develop hypotheses that take the form of computational models of this learning process, then test the models, comparing them to behavior and neural data in both humans and rodents.
You theorize that an important component of this process is ‘clustering’ experiences. What does this mean?
Imagine you have a favorite restaurant. You often get one specific dish there, say, massaman curry. You have a memory of how it tastes and how the dish is presented. You don’t actually remember the nuances of each time you ate it, because all these memories are clustered together. However, one day this dish is completely different, much more spicy, for instance. You might wonder if something went wrong, if they changed chefs or recipes. So you make a new cluster or memory. If in the future that dish is always spicy, you put those events in the new memory and use that to decide whether to order the dish again. If not, you can go back to updating the old memory.
What experimental evidence supports this type of clustered learning in humans?
We give people a task in which they look at a series of lines that gradually or abruptly change length. Then we ask them to recall the length of the first line they saw. When the line changes gradually, information is clustered in one big memory trace, so it’s hard to recall the length of the first line. But if the line suddenly gets much bigger in the middle of the experiment, our theory predicts the brain would start a new cluster. It would be easier to recall the length of the first line, because the lines the person sees after the big change — after they started a new cluster — would not interfere with that earlier memory. That’s what we see — people are better able to recall the first line when there is a big change in length during the experiment. None of this is instructed or conscious. People don’t necessarily think of the lines as two different memories. But if you ask them what they saw, they may indeed say, “First there were some short lines and then a bunch of long lines,” indicating two separate memory traces.
You have described this process as a sort of battle between learning and memory.
You can think of learning and memory as two competing forces. Animals can decide to learn by generalizing, grouping information into a cluster tied to one latent cause. Or they can memorize new information separately, keeping the old memory and creating a new cluster or latent cause.
With the spicy-curry example, I think the brain implicitly decides whether to learn that the curry here is now spicy, putting this experience in the old cluster and updating the expectation of spiciness for that memory cluster. Or it may decide it was a fluke and start a new cluster — memorizing separately the day when there was a hiccup and the curry was too spicy to eat — without updating general expectations for spiciness of massaman curry at this restaurant.
You’ve also shown that this type of clustering might be important in fear memories and perhaps in unlearning those types of memories.
If you train animals with an aversive experience, like a tone paired with a foot shock, they quickly learn to fear the tone after just one pairing. This has been a widely used model of learned phobias and PTSD. How do we change fear memory? If someone learned that something is very scary and they generalize that fear widely, how do we help them unlearn that association now that they’re in a safe environment?
We know from rats that if you play the tone without the shock many times, in a process known as extinction, animals fear the tone less and less. But that doesn’t seem to have changed the original fear memory. If you wait a couple of months and play the tone again, they are still afraid of the tone. If you test them in a new environment, they are still afraid of the tone. They learn that maybe the tone is less scary in a specific situation, but it’s still scary in general.
The general explanation for the failure of extinction to really change the underlying fear memory is that the animal doesn’t unlearn the tone-shock association, but rather learns a new association: between a tone and no shock. The two associations or memory traces compete over which controls behavior. So you didn’t really erase the old fear memory; you just created a new memory.
How can we use this idea to unlearn fear memories?
Based on our theory, we think we learn from similar things about similar things. The fact that there are no shocks during the extinction process makes it very different from the original training, so the brain makes a new memory (or association). But if we make the extinction process more similar to training, we might coax the rat to update the old memory. In our experiment, we gradually decreased the shocks, essentially ‘weaning’ the rats off the shock. We hoped that this would make extinction similar enough to the old experience that they would update the old memory. We compared these rats to those who had regular extinction (with no shocks at all), testing later for spontaneous recovery of fear. The rats that underwent gradual extinction no longer showed fear in response to the tone, even though they got more shocks in extinction than in training. Even with a reminder shock, they were still not afraid of the tone. The rats that underwent regular extinction were very afraid of the tone after the reminder shock.
Why does gradual extinction work?
Sam Gershman, when he was a graduate student in my lab, thought that perhaps animals learn something more sophisticated than simply the association between the tone and the shock. Maybe the rat learns the hidden structure in the environment — that there is a hidden variable, the experimenter, who causes both the tone and the shock.
The animal can then infer that one hidden variable or latent cause triggers a pairing of tone and shock, then a new latent cause delivers only tones with no shock. When we give the rat a reminder shock, we really give it a clue that the first latent cause is active again, and that’s why the animal freezes. Or, if we give the rat gradual extinction, the rat may infer that there is one latent cause responsible for everything in the experiment, and that it (thankfully) no longer causes shocks, so the rat is safe.
Why is the concept of clustering so important for understanding learning and memory?
Think about learning in the real world — there, experiences don’t come in trials, and stimuli are not simply tones or shocks. There is always a lot going on, and no two experiences are exactly alike. So the first thing we have to do is cluster experiences. We have to decide what is similar enough to be considered the same. We assume a dish in a restaurant will be similar the next day. But at a different restaurant, is it going to be the same? We are constantly making judgments based on similarity. These clusters determine the boundaries of learning; learning happens within clusters, not across them.
What I like about this phenomenon is how general it is. There is evidence for clustering in fear learning, perceptual learning and memory. I’m not surprised it’s such a general phenomenon. Our brain has to make this kind of decision all the time in order to be able to carry any information forward from one instance to another.
Are you trying to figure out how to use these ideas in clinical practice?
Not yet, but we would like to. In general, my lab is moving into the domain of computational psychiatry, a move that was inspired by this finding. We are trying to think about how models of learning, cognition, attention and memory that the community has developed in the last 20 years can help us understand and treat mental illness. I have been on sabbatical in Israel for the last year, immersing myself in clinical training. I spent time in a mental health hospital and took courses with psychiatrists and behavioral therapists.
Cognitive behavioral therapy is sort of like gradual extinction. It’s about activating an old association and then updating it. For instance, if you have social anxiety, you put yourself in a social situation and notice that, actually, it is not the case that everyone is laughing at you.
I’m also working on how ideas about learning, attention and memory can help tailor psychotherapy. Is someone generalizing too widely? Or maybe they are attending to the wrong thing and therefore not benefiting from the treatment? With computational models, we can fit parameters of models to each person, such as the width of the attention filter at each point in time. Maybe that can help therapists.
You’re involved in BiasWatchNeuro, a website funded in part by the Simons Foundation that tracks the diversity of the speakers at conferences in neuroscience. Why is this so important?
We have seen that this kind of tracking has made a difference. We did some statistics on conferences in our database as of the end of 2018, and we saw a marked improvement. At more and more conferences these days, the gender balance of speakers is at or above the field’s base rate of women, the percentage of women in that field. We would have thought this goes without saying, but previously that was not the case. There are still some conferences where that is not the case — I’ve seen conferences with 15 speakers and zero women. But fortunately those are becoming more rare.
There is a big sea change with the #MeToo movement, and a lot more attention to issues of gender bias in the press in general, from The New York Times to Science and Nature. But I like to think some of it is BiasWatch. For example, I heard of a book published by an academic press that said they needed more women authors for chapters in the book, otherwise they would end up on BiasWatchNeuro. I was so pleased that people think about it, even though BiasWatchNeuro only tracks conferences, not books. It doesn’t matter to me if it’s because of fear or some other reason, as long as they do the right thing. It really makes a difference for trainees; they see a different picture of who is a neuroscientist. I really hope it will trickle down.
Over the long term, we want to look at whether better representation of women at conferences improves the base rate in those fields. As women students and postdocs see more women as successful scientists, are they less likely to leak out of academia? BiasWatchNeuro has only been around for three years, so we haven’t seen a change yet. But we hope to see a bigger effect in 10 years.