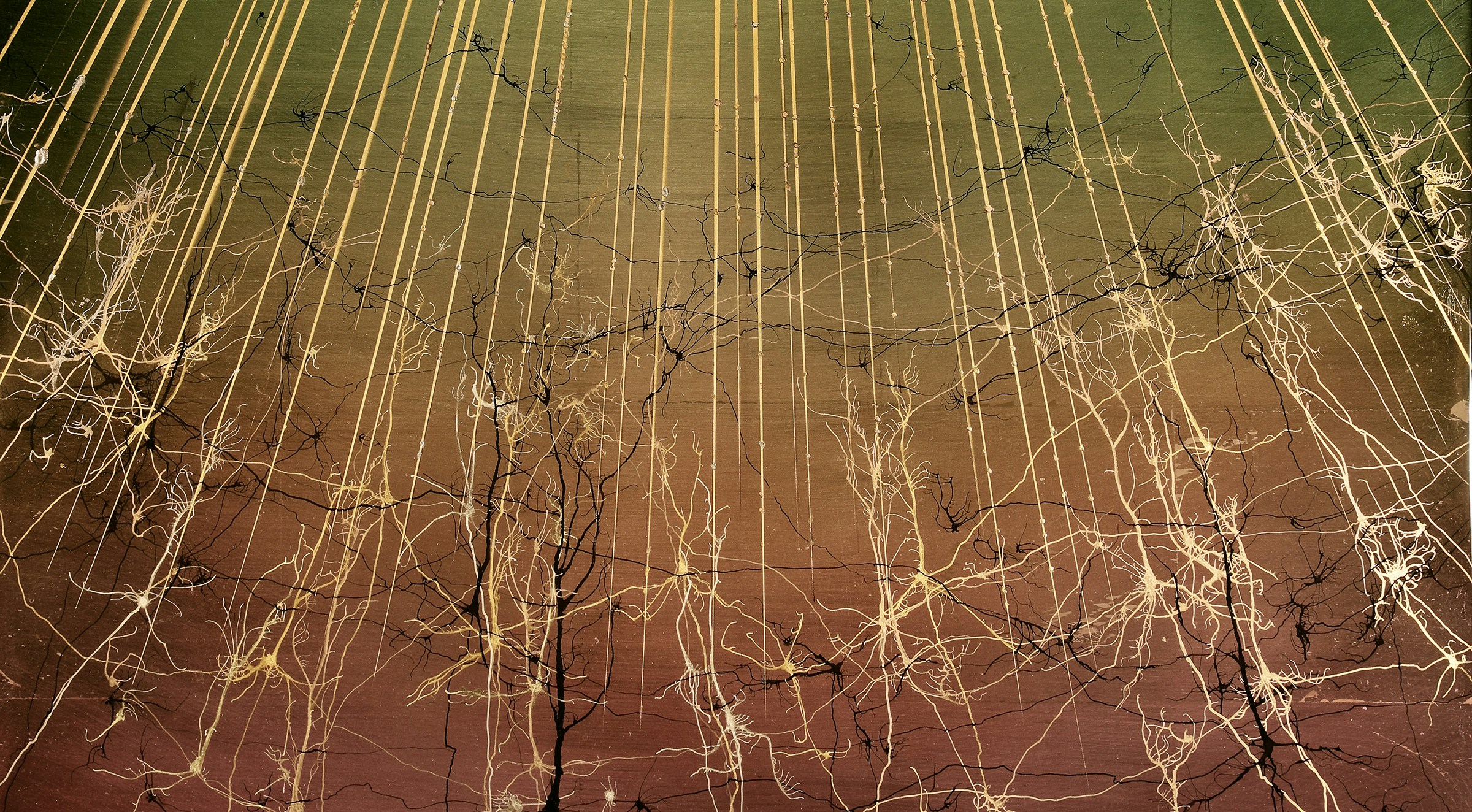
For Better Vision, Independent Neurons May Be Key
Imagine you’re in the woods trying to identify a species of bird. How well you execute this task depends on different factors — you’re more apt to spot the bird if you’re paying attention to the tree it’s sitting in or if you are an experienced birder and have seen this species frequently. What’s happening in your brain that makes it easier or harder to detect the bird, given the same sensory information?
New research suggests the answer lies in the behavior of groups of neurons in the visual cortex. Amy Ni, who is a fellow with the Simons Collaboration on the Global Brain (SCGB), and her collaborators found that better performance on a visual task is tightly linked to a drop in shared neuronal variability — how often neurons within a group fire together in response to the same visual stimulus. In other words, when neurons within a population act more independently of one another, the animal performs better. The research was published in Science in January 2018.
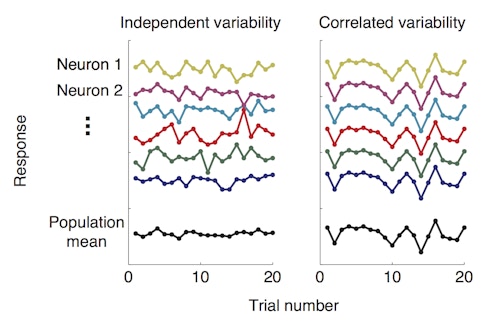
The finding supports a long-held theory that mechanisms that enhance vision, such as attention, do so by dampening the correlations among neurons. According to the idea, put forth by researchers including Marlene Cohen, a neuroscientist at the University of Pittsburgh and an SCGB investigator, this in turn increases the amount of visual information that a group of neurons can encode, enabling the animal to see better. By contrast, if a group of neurons is highly correlated, each individual neuron provides little additional information about what the animal sees.
“The idea Marlene has proposed for some time is that this change in correlation is one of the main things the brain does to improve the quality of the signal,” says Tony Movshon, a neuroscientist at New York University and an SCGB investigator.
Previous research by Cohen and others has shown that both attention and practice, or ‘perceptual learning,’ can dampen correlated variability — how much the activity of individual neurons in a group tend to fluctuate together. But the new study is the first to tie correlated variability so tightly to how well an animal sees.
“This paper shows that you can really predict how well an animal will do by looking at the correlations on that particular day,” says Adam Kohn, a neuroscientist at Albert Einstein College of Medicine in New York and an SCGB investigator. “That’s true across multiple domains — attention and learning — suggesting it’s a general mechanism.”
Different timescales:
In the new study, Ni, Cohen and their collaborators analyzed the effects of both attention and perceptual learning in the same animals as they performed a single task: identifying the orientation of a striped pattern. “It’s an impressive technical feat,” says Kohn, who collaborates with Cohen but was not involved in the study.
Ni, a postdoctoral researcher in Cohen’s lab, wanted to study these two processes together because they both improve performance but on very different timescales — attention operates in milliseconds, whereas perceptual learning takes days to months. “If both attention and perceptual learning use the same brain mechanism, that tells us it must be an important signature of brain activity for improving vision,” Ni says.
She found that not only do both attention and perceptual learning weaken correlated variability, they do so in a strikingly similar fashion. “They are slap-bang on top of each other,” says Movshon, who was not involved in the study. “It’s a nice demonstration that this is a core function of sensory cortex that is manipulated by several different things in the same way.”
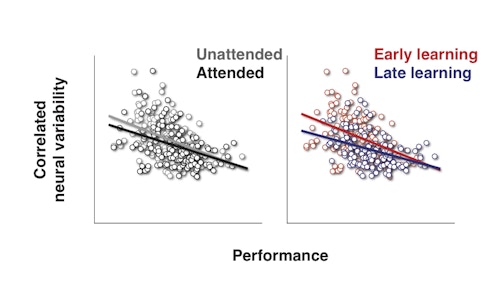
Ni and her collaborators next plan to test whether other ways of improving vision use the same mechanism. They will treat animals with medications prescribed for attention disorders, such as methylphenidate (commonly marketed as Ritalin), to see if the drug also changes correlated variability. “Are the drugs using this common mechanism, altering how independently neurons encode information?” Ni says.
The answer could have important implications for how to treat attention and other cognitive conditions, Cohen says. “Maybe we should look for drugs that have that specific effect on variability in neural populations,” she says. “It might help us understand the underlying mechanism for how these drugs work.”
Optimal decoding:
The new findings contribute to an ongoing debate over the role that correlated activity plays in information processing. These correlations can be divided into specific forms, and a theoretical study published in 2014 found that only a very specific subset limit how much information a population of neurons can encode.
That raised the possibility that this measure may be a side effect rather than the mechanism by which attention and learning improve vision. However, the Science study found that an overall measure of correlation, rather than a specific subset, predicts performance. In other words, “mean correlations are a functionally important variable,” Kohn says. Such a conclusion does not invalidate the theoretical framework, he says. Instead, “it suggests there is a great deal we don’t know about how population responses are used in the animal.”
One potential limitation of the theoretical studies is that they employed a specific algorithm — known as an optimal decoder — to extract information from visual cortex neurons about what the animal was looking at. But in reality, the brain doesn’t make decisions based on this set of information. Rather, neurons in the visual cortex send information to different brain areas, which perform additional computations that ultimately drive our reaction to what we see. “There is mismatch between the clean, elegant theoretical framework and the way population responses are used in the brain,” Kohn says.
Indeed, Cohen and Ni’s work suggests animals don’t decode information optimally. “Instead, animals appear to be suboptimal in a way that makes changes in correlated variability have an outsized impact on the behavior,” Cohen says. “The key question is not how attention or learning affects the amount of information that’s there if you could decode optimally but how they affect the information that’s actually used to guide behavior.”
Cohen will attempt to address this question in her new SCGB project, a collaboration with Kohn and several theorists, including SCGB investigator Alexandre Pouget, who authored the 2014 theoretical paper. To better understand how the brain uses sensory information to guide behavior, the researchers will study brain activity underlying more complex behaviors and record from multiple brain areas simultaneously. “We plan to spend the next four to five years duking that out,” Cohen says.